The Ultimate Interface for Drug Discovery: How Spatial Computing and AI Agents Are Transforming How We Understand Molecules
July 31, 2024
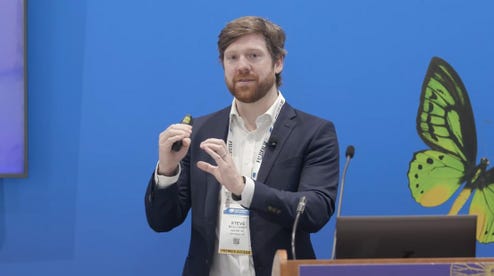
Steve McCloskey, founder and chief executive officer, Nanome
McCloskey began his presentation by sharing an artificial-intelligence (AI)–generated image of the future of AI-supplemented drug discovery. Although the picture suggested that such a future will be run primarily by machines with minimal human involvement, McCloskey explained that the more immediate future instead will involve the evolution of existing tools. The incorporation of AI and virtual reality (VR) into drug-discovery tools is not likely to replace current technologies, but rather to enhance them. For example, smart devices were developed as an enhancement of computer-monitor setups; scientists still use conventional setups in drug-discovery activities, even in advanced fields such as computational chemistry and protein-structure analysis.
One goal of using AI and VR tools is to analyze three-dimensional data in ways that were not possible before. Mixed-reality experiences, such as that provided by the Varjo XR-3 interface, will enable users to analyze protein structures, design new chemicals, and derive key insights for drug candidates and process efficiency. Such tools offer predictive structures for early decision-making and understanding of potential variations and mutations.
According to McCloskey, the evolution of such tools should focus on improving human interfaces and collaboration. Although AI-supported approaches can enhance data collection compared with traditional research and development (R&D) workflows, the human users must make decisions based on the data provided. Interfaces tailored to human decision-making will be crucial for successful R&D activities. Similarly, for companies with local and international collaboration activities, AI and VR tools will assist in bringing all members of a team together in real time, even when they reside in different countries and time zones. Conducting meetings in that way would keep team members aligned when discussing drug candidates to take forward or analyzing binding pockets on proteins, for example. Novartis uses VR headsets to hold regular meetings. In one case, doing so shaved four months off of a six-month process. Such time savings can accelerate the path to clinical trials and decrease time to market, which leads to better outcomes for patients.
The current industry focus for AI is on automated scientific methods and algorithms — e.g., de novo molecule generation, protein-structure prediction, retrosynthesis, and accelerated computer modeling. However, McCloskey explained that an overlooked opportunity in AI advancement is amplifying human performance. AI tools that automate workflows, simplify data analysis, orchestrate new tasks, and inspire new insights for drug development can contribute to increased human understanding of proteins and molecules, therefore improving process efficiency and increasing cost savings. “You can’t just treat AI as a black box where you don’t know what’s going on in it, and eventually you hope that it comes up the next billion-dollar drug,” McCloskey said. Noting that many people consider the next step in AI development to be fully automated and/or robotic processes, he again emphasized that AI tools require human intervention to make a positive impact on drug development.
AI tools and foundational models have their own challenges, however. Training data often represent snapshots from specific timepoints rather than real-time occurrences. Models sometimes “hallucinate” text and mathematical logic, meaning that they cannot rationalize a request and instead move onto the next token of response, generating inaccurate or nonsensical outputs. Currently, AI tools cannot be used for complex scientific questions and thus require human intervention for interpretation of data and results.
McCloskey concluded by discussing the democratization of computational tools and data. Open-source tools have gained popularity with large pharmaceutical companies despite the challenges associated with learning how to operate them and installing their infrastructures. Developers must continue to collaborate both in drug discovery and AI-tool enhancement to bring drugs to market, improve disease understanding, and design treatment regimens. Nanome currently is developing the Molecular Analysis and Reasoning Agent (MARA) AI program, which will function as a “scientific research assistant” for protein analytics.
Watch Online
See full presentations online at https://bioprocessintl.com/category/bpi-theater/bpi-theater-bio-2024
You May Also Like