A Scale-Up/-Down Platform for Fast-Paced Upstream Process Development of rAAV-Based Gene TherapiesA Scale-Up/-Down Platform for Fast-Paced Upstream Process Development of rAAV-Based Gene Therapies
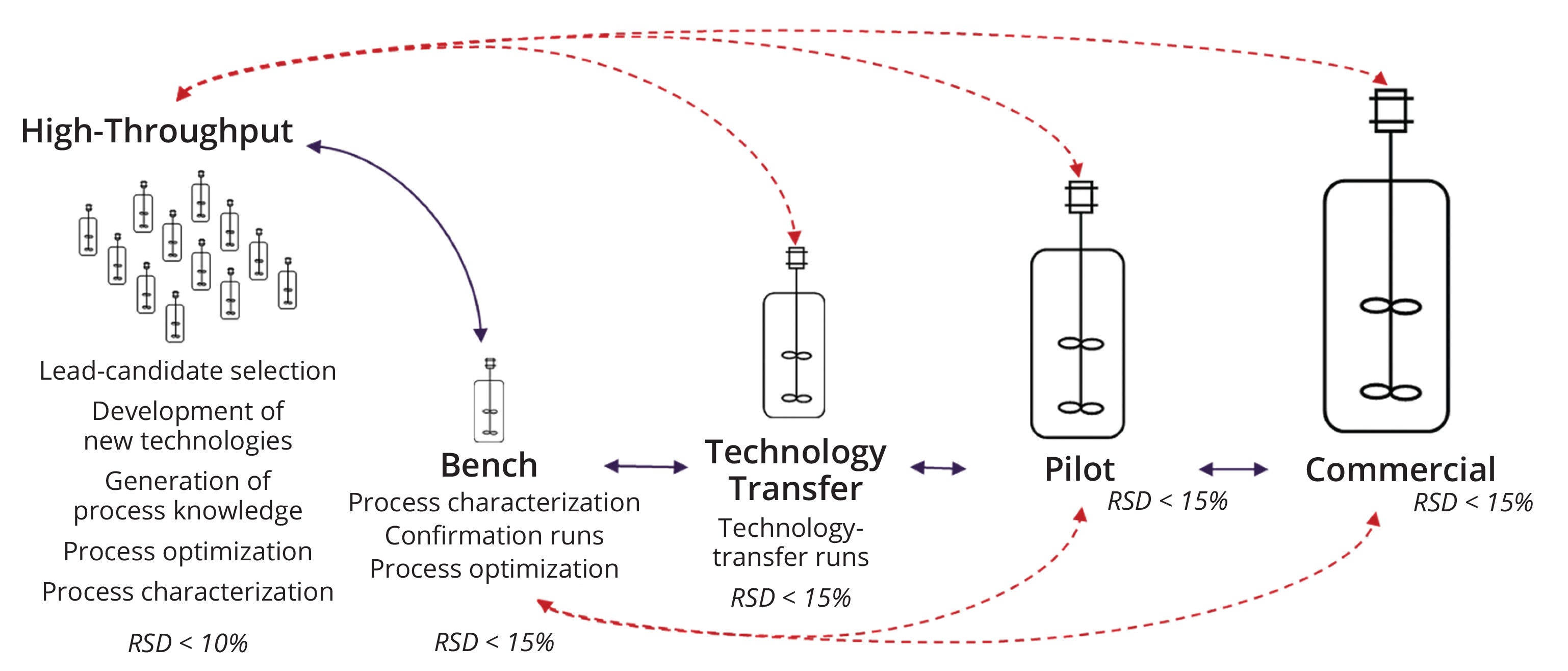
Graphical Abstract: We discuss our development of a robust, fast-paced, and high-throughput (HT) process-development platform for production of recombinant adenoassociated virus (rAAV) vectors using insect cells and a baculovirus expression vector system (BEVS). Based on theoretical regime analysis, we redesigned upstream operations to generate bench and HT scale-down models that were representative of a large-scale process. Data collected during experimental validation showed that productivity levels and measured critical quality attributes (CQAs) matched across scales, demonstrating scalability from HT to commercial scale. Our findings can facilitate process characterization for rAAV production processes. As shown below, our data also suggest the possibility of bypassing intermediate scales during process development, effectively shortening timelines and decreasing costs for such activities. Solid lines in the image represent a traditional, linear development pathway, whereas the dashed, red lines indicate our propose development paths (RSD = relative standard deviation).
The field of bioprocess development has witnessed remarkable advancements in recent years driven by increasing demand for biopharmaceuticals, including gene-therapy products such as recombinant adenoassociated virus (rAAV) vectors. To date, global regulatory bodies have approved several rAAV-based gene therapies, and multiple clinical trials are ongoing as forecasts project significant growth over the next five to 10 years (1).
The field of rAAV production continues to be dominated by platforms using human embryonic kidney (HEK) and insect cells. For instance, uniQure’s proprietary platform is based on insect-cell production and a baculovirus expression vector system (BEVS) (2–5). As the gene-therapy clinical pipeline expands, ensuring efficient, cost-effective, and scalable processes for viral-vector production becomes critical (1). Central to that endeavor are scale-down models and fast-paced scale-up.
Scale-down models are small-scale representations that are designed to mimic key process parameters, conditions, and challenges encountered at production scale (6). Such models are instrumental in providing insights into process performance, identifying potential bottlenecks, and enabling process improvements without the need for large-scale, resource-intensive trials. Thus, scale-down models have become an indispensable tool in the biotechnology industry for developing, understanding, optimizing, and validating bioprocesses (7, 8).
Multiple bioreactor systems have demonstrated consistent scalability across bench, pilot, and commercial production scales for biopharmaceuticals, including cell and gene therapy (CGT) products (9–12). The availability of such equipment shows that scaling up from benchtop to commercial manufacturing is not only possible, but also well-established in the industry.
Fast-paced scale-up refers to bridging the gap between laboratory-scale experiments and full-scale production in the shortest time frame. Several biopharmaceutical companies have adopted that philosophy as a result of implementing learnings from the successful deployment of SARS-CoV-2 vaccines in record times (13, 14). A fast-paced approach is possible largely due to abundant internal know-how, platform-based approaches (7, 15), and high-throughput experimentation and analytics.
In that respect, high-throughput miniaturized bioreactors offer a cutting-edge solution to the problem of data generation toward expediting process development. Herein, we explore the pivotal role of such systems in scaling up processes, optimizing gene-therapy production, and streamlining biomanufacturing processes based on insect cells. Our objective is to establish a representative scale-down model of our current production bioreactor to support a platform capable of hastening commercial manufacturing of potentially lifesaving and life-transforming therapies. We propose a relatively unconventional approach to process development by providing data that support the possibility of bypassing small-scale studies and scaling up directly from minibioreactors to pilot/commercial scales for rAAV production.
Materials and Methods
Regime Analysis: In the context of gene-therapy scale-up/-down, the regime-analysis approach serves as a fundamental tool for assessing and characterizing the behavior of bioprocesses at different scales, mainly the production-scale bioreactor, which is the target operation to be studied (16–18). This analytical approach facilitates comprehension of both critical time constants and the influences of different parameters and operating conditions on process performance. It guides efforts to scale down processes into benchtop and high-throughput bioreactors while helping process engineers to select optimal operating spaces at commercial and intermediate production scales.
Several critical time constants are used during model characterization to ensure accurate representation of bioreactor performance at a larger scale (8). Key parameters to address during model design include power input per unit volume (P/VL), impeller-tip speed (vtip), superficial gas velocity (vsg), and rate of overlay gas flow (fov). We conducted a literature survey to gather sufficient empirical correlations for performing a regime analysis, and we selected the most appropriate time constants for model design and testing (4, 8–12, 16–36).
Scaling up cell-culture processes in stirred-tank bioreactors (STRs) requires careful consideration of equipment capabilities in terms of mass, temperature, and momentum transfer — which can be characterized using the well-documented oxygen mass-transfer coefficient (kLa), heat-transfer coefficient (U), and mixing time (θm), respectively (19–21). In addition, process engineers need a fair understanding of a host cell line’s biological properties, such as its shear resistance, death rate (kD), and growth rate (µmax) (22–24, 26). One consideration for processes involving BEVs is that host cells are known to undergo physiological changes after baculovirus infection (4, 25, 27, 37). An additional complication for scale-up design is that many of the variables described above have interdependencies (Figure 1). For example, media composition influences cell performance, which in turn can affect STR capacity for mass transfer.
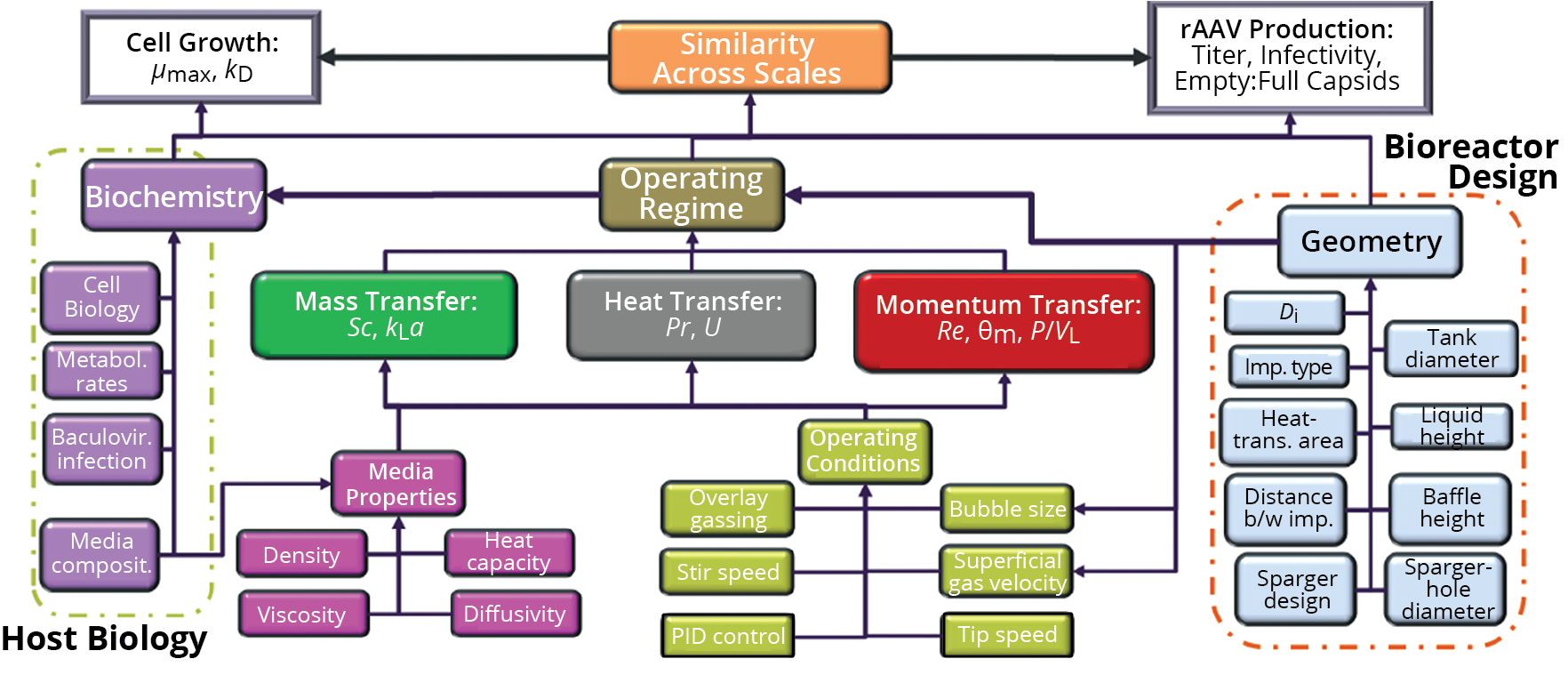
Figure 1: A regime analysis mapping potential interactions among the main variables that influence cell-culture performance during production of recombinant adenoassociated virus (rAAV); Di: impeller diameter, imp.: impeller, kD: cell death rate, kLa: oxygen mass-transfer coefficient, P/VL: power per unit volume, PID: proportional–integral–derivative, Pr: Prandtl number, Re: Reynolds number, Sc: Schmidt number, θm: mixing time, U: heat-transfer coefficient, µmax: cell growth rate
Cell Expansion in Shake Flasks: The insect cell line used throughout this study was cultivated in single-use shake flasks with working volumes ranging from 125 mL to 2 L. We applied standard conditions reported in literature (4, 5, 38, 39).
Cell Growth and rAAV Production in STRs: We performed different studies to establish STR operating conditions for our scale-down models. Specifically, we investigated settings for dissolved-oxygen–concentration (%DOsat) cascade and proportional–integral–derivative (PID) control parameters to match performance in terms of cell growth and rAAV production across scales. Published experimental conditions for growing insect cells and producing rAAV in STRs served as a starting point for process optimization (38–40). We assessed cell growth experimentally at high-throughput (<0.5 L), benchtop (<5 L), and technology-transfer scales (<100 L). Different iterations in operational parameters were selected based on our earlier regime analysis, with data obtained at pilot scale (>100 L) serving as our target for optimization. After selecting successful conditions, we challenged the rAAV production process at all scales to validate its scalability, demonstrating similar performance in the form of statistically comparable (95% confidence in a t test) product yield and main product critical quality attributes (CQAs), namely, infectivity and empty to full capsid (E:F) ratios.
Results and Discussion
Selection of scale-up/-down factors is a critical aspect of bioprocess development because that process ensures successful transition from systems such as high-throughput (milliliter-scale) and benchtop (liter-scale) bioreactors to vessels of larger scales (e.g., hectoliter scale). During technology transfer from benchtop- (<5 L) to commercial-scale (>200 L) rAAV production, determination of biological and operational factors becomes paramount.
We needed data about STR geometry (e.g., tank diameter and impeller number and diameter), physiochemical properties (density and heat capacity), operating conditions (stirring and aeration rates), and cell-inoculation density to compare our operational regime across scales. Key process parameters such as oxygen mass transfer and power consumption were calculated using empirical correlations that we found during our literature survey. Our choice of scaling factors was guided, on one hand, by the theoretical assessment provided by the regime analysis and, on the other, by experimental validation. Parameter selection hinged on results from different experimental iterations, and experiments were guided largely by findings from the regime analysis and learnings obtained during performance matching across scales (based on cell growth and rAAV production). Although we did not leverage such tools, we encourage use of artificial intelligence (AI) and machine learning (ML) to speed up scale-down endeavors and decrease the experimental workload — e.g., by using approaches outlined in Alavijeh et al. (41).
That said, empirical confirmation of operational conditions and predictions from the regime analysis was an essential part of our process. For instance, experimental results highlighted concerns relating to bioreactor control, specifically the selection of nonscalable PID control parameters. Consideration of such factors was critical to ensuring performance matching (Figure 2). We performed PID tuning and validation using a mix of empirical testing and traditional tuning methods and modeling, as discussed by Harcum et al. (42).
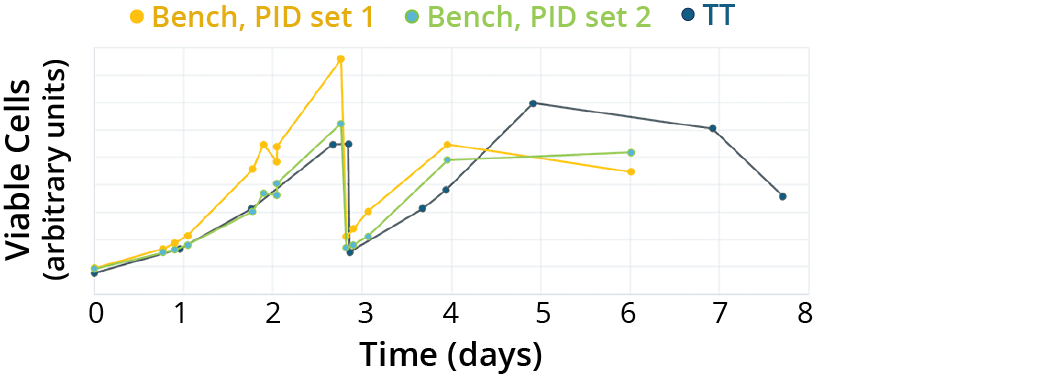
Figure 2: Influence of proportional–integral–derivative (PID) parameters on cell expansion; the trends below show the critical role of PID parameters during performance-matching experiments between bench- (yellow and green lines) and technology-transfer (TT)–scale bioreactors (black line). The bench-scale bioreactor operated with PID set 2 (green line) more closely matched the performance of the TT bioreactor than did the reactor using PID set 1 (yellow line).
Cell Proliferation at Different Scales: After selecting scalable operational conditions, we monitored insect-cell growth during STR cultivation with the goal of demonstrating similar performance across scales, measured as a statistically similar growth rate (95% confidence interval in a t test) (Figure 3). The obtained data suggest that specific growth rates were calculated with <5% deviation between scales, confirming that the selected operating conditions provided a similar environment for cell proliferation.
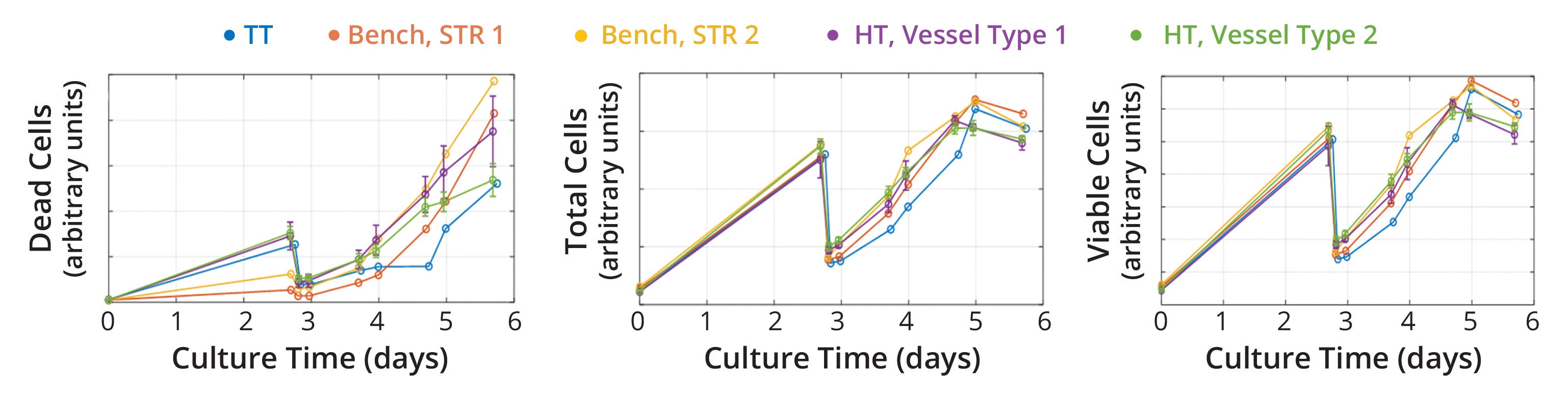
Figure 3: Cell-growth profiles across scales; similar growth rates were achieved across technology-transfer (TT), bench, and high-throughput (HT) scales when using stirred-tank bioreactor (STR) operational conditions that were selected based on regime analysis and empirical testing. For HT-scale experiments, different vessel geometries underwent testing and showed no impact on cell proliferation.
We observed some minor differences in dead-cell counts. In practical terms, those differences did not influence cell growth and rAAV production, but we performed additional experiments to identify potential root causes. We attribute the differences in cell-death profiles mainly to the presence of a microsparger in the larger-scale cultivation vessel, as opposed to an L-shaped sparger in the benchtop and high-throughput vessels (11).
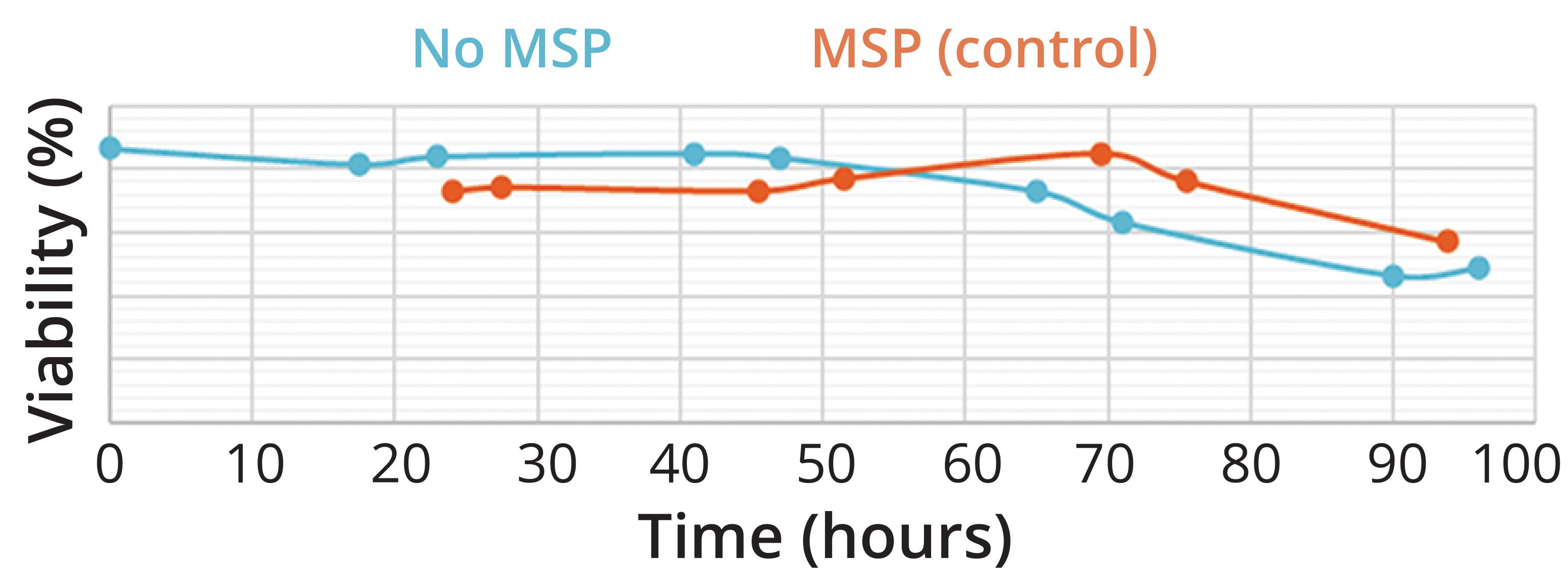
Figure 4: Cell-viability profile in the absence and presence of microsparger (MSP)–based control of dissolved oxygen. Adding O2 through a ring sparger generated cultures with lower cell-viability levels than did using MSP control.
Small, high-energy bubbles are known to influence cell-viability levels during cultivation (43–45). Figure 4 shows the experimental demonstration confirming our hypothesis that differences in the dead-cell counts can be attributed to hydrodynamic stress generated when oxygen is added through a microsparger for %DOsat control. The higher viability observed with the microsparger-equipped vessel (the orange line in Figure 4) is associated with cell lysis due to hydrodynamic stress. Dead cells are unable to repair their biological structures, making them prone to lysis and less detectable than needed using traditional cell-counting methods. On the other hand, the vessel equipped with a ring sparger showed lower cell viability. Our data suggest that a ring sparger kept dead cells intact because it exerted lower hydrodynamic stress than did the microsparger. These results accord with literature reporting that small bubbles are linked to high-energy explosions that result in cell damage and concomitant cell death (46).
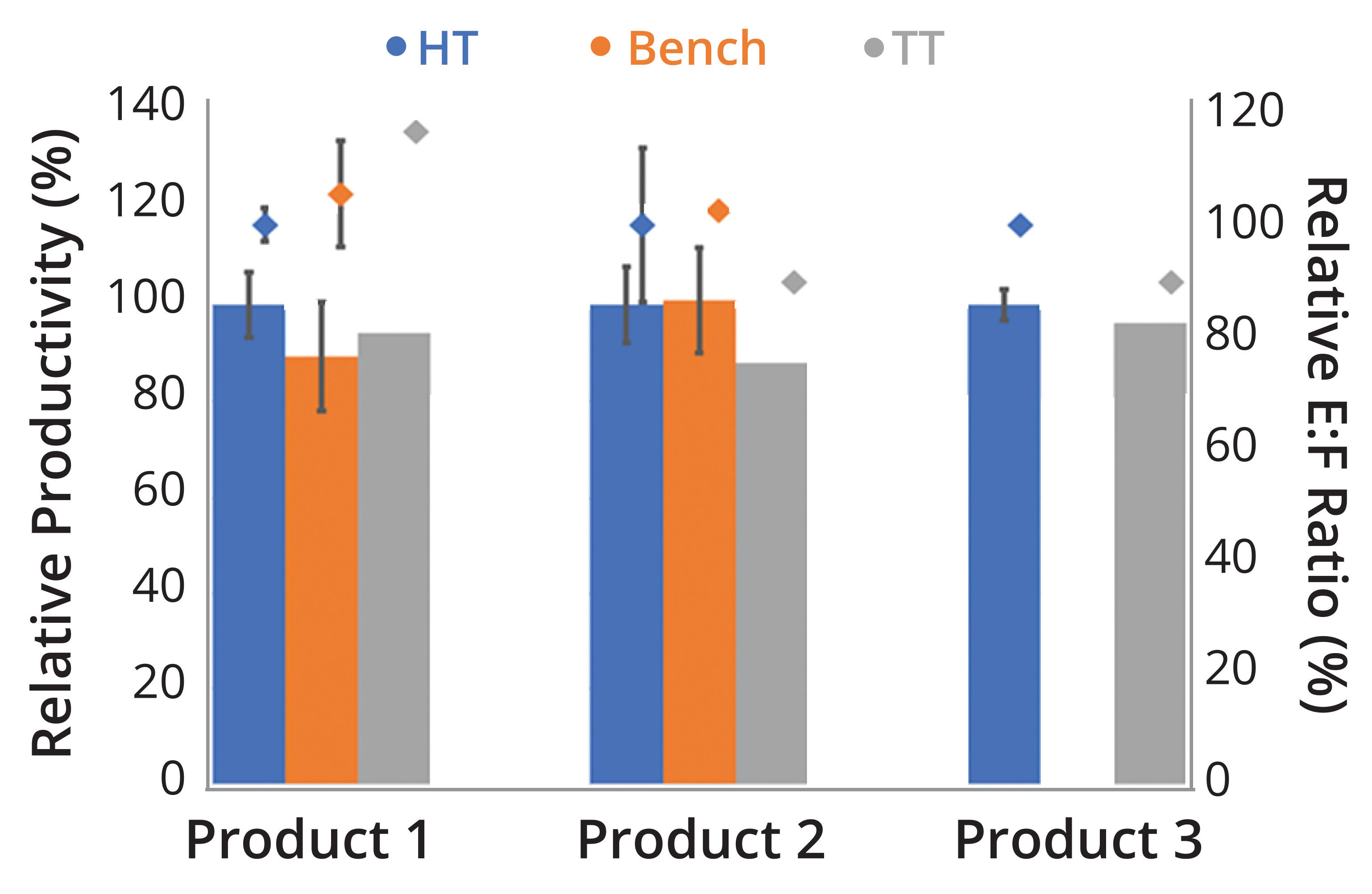
Figure 5: Performance comparison of three products based on recombinant adenoassociated virus (rAAV) vectors; our experimental data suggest nonsignificant variability obtained across high-throughput (HT), bench, and technology-transfer (TT) scales. (E:F = ratio of empty to full rAAV capsids)
rAAV Production at Different Scales: After implementing operational conditions that matched cell-proliferation levels across the small (high-throughput, benchtop, and technology-transfer) and pilot/commercial scales, we further challenged our scale-down model to investigate whether production of different rAAV targets would result in similar performance. Figure 5 plots relative values for productivity (viral genomes per milliliter, vg/mL) and E:F ratios for three rAAV products processed at high-throughput, benchtop, and technology-transfer scales, with results from the high-throughput processes set at 100%. We observed nonsignificant variability (95% confidence, t test) across scales.
Similarly, we tracked the performance of a single rAAV product from high-throughput to pilot/commercial scale, assessing relative values for productivity (vg/mL), E:F ratios, and infectivity (genome copies per infectious unit, gc/IU) with high-throughput values set as 100%. Results were consistent with our previous data, with nonsignificant variability across all scales (Figure 6). Thus, we confirmed that operational conditions selected for the different small-scale bioreactors accurately represented those for the pilot/commercial scale.
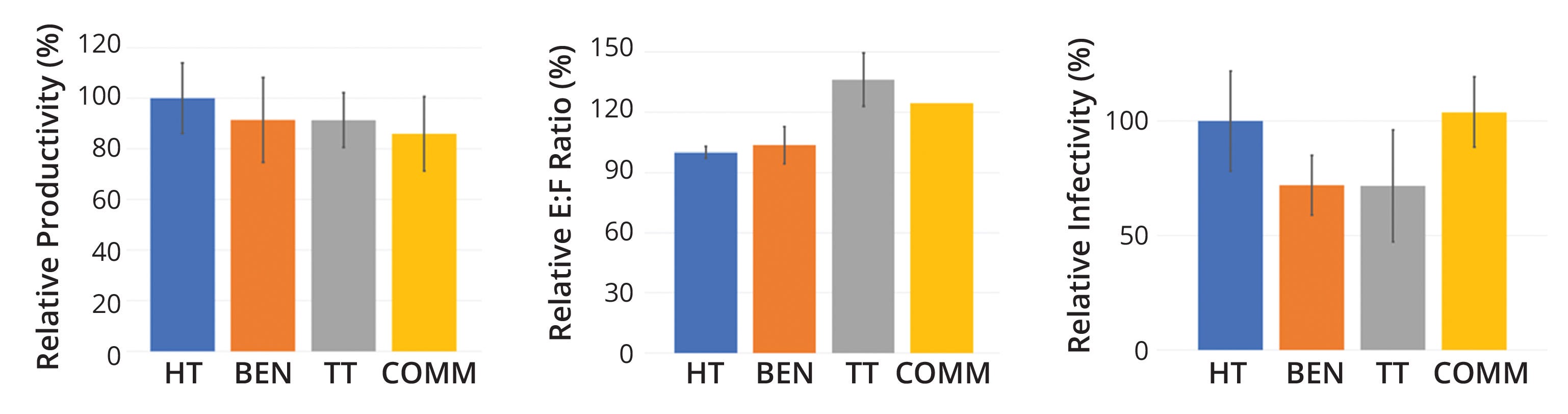
Figure 6: Performance comparison across scales for the same recombinant adenoassociated virus (rAAV) product; relative productivity (LEFT), relative ratios of empty to full rAAV capsids (E:F) (CENTER), and relative infectivity levels (RIGHT) all showed nonsignificant variability across commercial (COMM), technology-transfer (TT), bench (BEN), and high-throughput (HT) scales.
The above results enable us to propose a new development pathway toward shortening timelines for rAAV production. Traditional approaches rely on small-scale experiments followed by confirmatory studies in different iterations at a larger scale. In our proposed workflow, initial studies can be performed at a high-throughput scale, then move directly to technology-transfer scale and further to pilot testing. Bypassing bench-scale and technology-transfer confirmation studies could shorten the development pathway by up to 20% — from 20 to 16 weeks — with significant decreases in development costs (Figure 7).
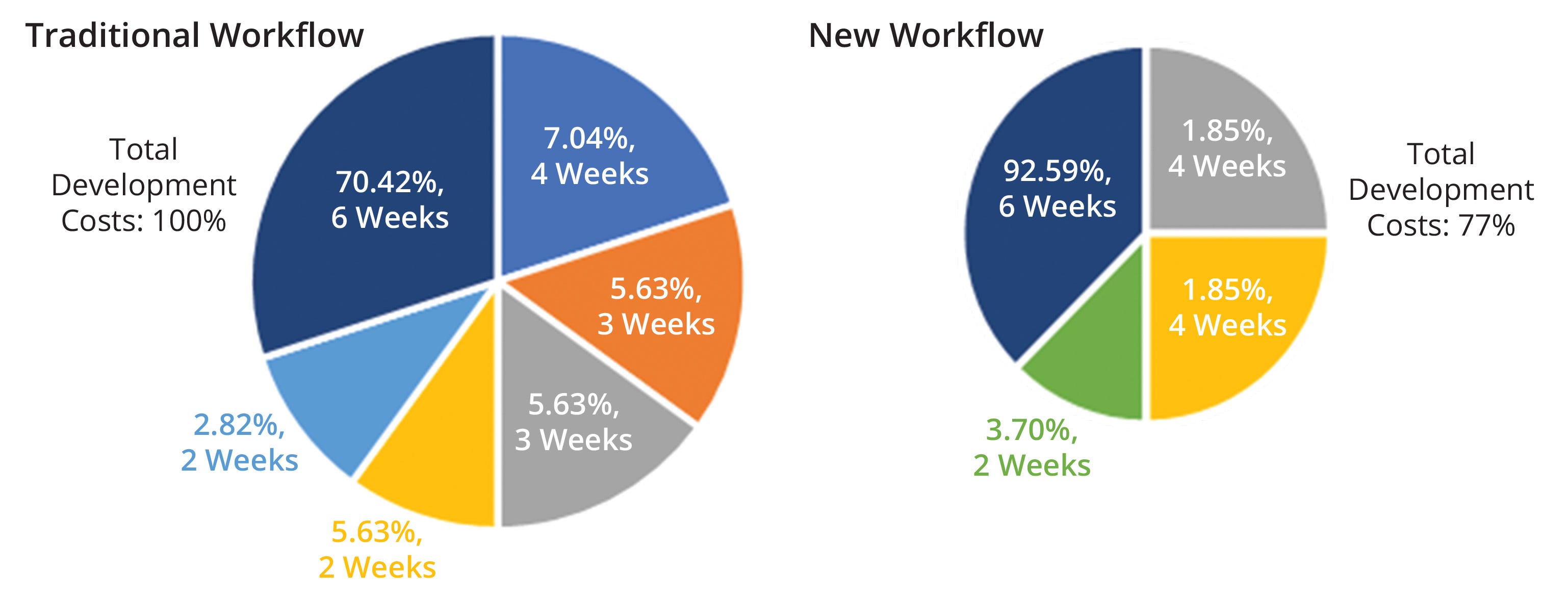
Figure 7: Technoeconomic assessment of a traditional and proposed workflow for production of recombinant adenoassociated virus (rAAV) vectors; the size of each chart reflects the total development costs of its associated workflow. Each pie-chart fraction represents a work package related to developing a new rAAV product. The weeks listed refer to the time needed per work package, and listed percentages refer to the cost of a work package relative to total development costs. The new workflow represents a cost savings of 23%.
Conclusions
Typical challenges to scaling cell-culture processes include defining and understanding the key phenomena that match performance across scales. Our work suggests that a good starting point is applying a combination of rational and empirical approaches. On one hand, the classical tool of regime analysis and other mathematical models helped us to constrain the design space of our cell-culture operation. On the other hand, empirical approaches for tuning PID controllers and matching bioreactor performance were essential to the endeavor of developing and validating a scale-down model based on the performance of a large-scale STR.
The data presented suggest that operational conditions selected for high-throughput and bench scales achieved similar outcomes in larger-scale (technology-transfer and pilot/commercial) bioreactors, as shown by the statistically insignificant variability in productivity levels, E:F ratios, and infectivity levels of different rAAV products.
We believe that developing well-characterized and validated scale-down models at high-throughput scale (mL) will be essential to establishing a platform approach to process development and to accelerating time to market for rAAV products. The possibility of testing many conditions in a design-of-experiments (DoE) format in combination with empirical validation of scalability — and using no additional studies at intermediate bioreactor scales — would account for much of the substantial reductions in timelines and development costs.
Acknowledgments
Experimental support, cell-growth data, and rAAV production data at technology-transfer scale were kindly provided by Sara Botas Sanmartin, Jeroen Verbruggen, Joyce Ijspelder, Maaike Steenkamp, Marsha Haneveld, Giorgio Rainone, Milja Pesic, Rupali Desai, Yang Jiang, and Tangir Ahamed, all part of the process development team of uniQure B.V. in Amsterdam, the Netherlands. Pilot-scale and (semi)commercial data were shared by the manufacturing science and technology (MSAT) team at uniQure Inc. in Lexington, MA, USA. Monika Golinska, Nasser Sadr, and their teams supported scalability assessment with analysis of the rAAV product and process intermediates.
Author Contributions
Pavlištová performed data analysis and drafted the manuscript. Correia and Molina Gil performed critical experiments and supported them with data analysis. Van de Waterbeemd, Ljubovic-Couteau, and Streefland challenged and reviewed the experimental data and manuscript content. Cueto-Rojas designed experiments, challenged and reviewed data, and drafted this manuscript.
References
1 Jiang Z, Dalby PA. Challenges in Scaling Up AAV-Based Gene Therapy Manufacturing. Trends Biotechnol. 41(10) 2023: 1268–1281; https://doi.org/10.1016/j.tibtech.
2 Guan J-S, et al. Process Improvement of Adeno-Associated Virus Production. Front. Chem. Eng. 4, 2022: 830421; https://doi.org/10.3389/fceng.2022.830421.
3 Lubelski J, Hermens W, Petry H. Insect Cell-Based Recombinant Adeno-Associated Virus Production: Molecular Process Optimization. BioProcessing J. 13(3) 2014: 6–11; https://doi.org/10.12665/J133.LUBELSKI.
4 Zitzmann J, et al. Process Optimization for Recombinant Protein Expression in Insect Cells. New Insights into Cell Culture Technology. Gowder SJT, Ed. IntechOpen: London, UK, 2017; https://doi.org/10.5772/67849.
5 Joshi PRH, et al. Advancements in Molecular Design and Bioprocessing of Recombinant Adeno-Associated Virus Gene Delivery Vectors Using the Insect-Cell Baculovirus Expression Platform. Biotechnol. J. 16(4) 2021: 2000021; https://doi.org/10.1002/biot.202000021.
6 ICH Q11. Development and Manufacture of Drug Substances (Chemical Entities and Biotechnological/Biological Entities). International Conference for Harmonisation of Technical Requirements for Registration of Pharmaceuticals for Human Use: Geneva, Switzerland, 1 May 2012; https://database.ich.org/sites/default/files/Q11%20Guideline.pdf.
7 Nilapwar S, et al. Leveraging Platform and Process Characterization Data To Accelerate CGT Validation and Commercialization. BioPhorum Development Group: Sheffield, UK, 2024; https://www.biophorum.com/download/leveraging-platform-and-process-characterization-data-to-accelerate-cgt-validation-and-commercialization.
8 DiCesare C, et al. Development, Qualification, and Application of a Bioreactor Scale-Down Process: Modeling Large-Scale Microcarrier Perfusion Cell Culture. BioProcess Int. 14(1) 2016: 18–29; https://www.bioprocessintl.com/expression-platforms/development-qualification-and-application-of-a-bioreactor-scale-down-process-modeling-large-scale-microcarrier-perfusion-cell-culture.
9 Sanderson TP, et al. Scalability Comparison Between 50 and 500 Liter Stirred Tank Bioreactor for Production of rAAV Viral Vector. Cell & Gene Ther. Ins. 7(9) 2021: 1025–1033; https://doi.org/10.18609/cgti.2021.131.
10 Kaiser SC, et al. Scaling-Up of an Insect Cell-Based Virus Production Process in a Novel Single-Use Bioreactor with Flexible Agitation. Chem. Ingen. Technik 94(12) 2022: 1950–1961; https://doi.org/10.1002/cite.202200103.
11 De Wilde D, et al. Superior Scalability of Single-Use Bioreactors. BioProcess Int. 12(8s) 2014: s14–s19; https://www.bioprocessintl.com/single-use/superior-scalability-of-single-use-bioreactors.
12 Zangi A, et al. Increased Efficiency and Product Quality with the UniVessel Single-Use Bioreactor for CHO Fed-Batch Cultures (application note). Sartorius Stedim: Göttingen, Germany, 20 November 2017.
13 Chavda VP, et al. Fast-Track Development of Vaccines for SARS-CoV-2: The Shots That Saved the World. Front. Immunol. 13, 2022: 961198; https://doi.org/10.3389/fimmu.2022.961198.
14 Brown P, et al. “It Seems Impossible That It’s Been Made So Quickly”: A Qualitative Investigation of Concerns About the Speed of COVID-19 Vaccine Development and How These May Be Overcome. Hum. Vacc. Immunother. 18(1) 2022: 2004808; https://doi.org/10.1080/21645515.2021.2004808.
15 Bradl H, et al. Platform Approach Speeds Process Development. BioPharm Int. 29(4) 2016: 20–25; https://www.biopharminternational.com/view/platform-approach-speeds-process-development.
16 Sweere APJ, Luyben KCAM, Kossen NWF. Regime Analysis and Scale-Down: Tools To Investigate the Performance of Bioreactors. Enzym. Microb. Technol. 9(7) 1987: 386–398; https://doi.org/10.1016/0141-0229(87)90133-5.
17 Pollard DJ, et al. Scale-Up of a Viscous Fungal Fermentation: Application of Scale-Up Criteria with Regime Analysis and Operating Boundary Conditions. Biotechnol. Bioeng. 96(2) 2007: 307–317; https://doi.org/10.1002/bit.21112.
18 Zlokarnik M. Dimensional Analysis and Scale-Up in Chemical Engineering. Springer-Verlag Berlin: Heidelberg, Germany, 1991; https://doi.org/10.1007/978-3-642-76673-2.
19 van ’t Riet K, Tramper J. Basic Bioreactor Design. CRC Press: Boca Raton, FL, 1991.
20 Garcia-Ochoa F, Gomez E. Bioreactor Scale-Up and Oxygen Transfer Rate in Microbial Processes: An Overview. Biotechnol. Adv. 27(2) 2009: 153–176; https://doi.org/10.1016/j.biotechadv.2008.10.006.
21 Varley J, Birch J. Reactor Design for Large Scale Suspension Animal Cell Culture. Cytotechnology 29(3) 1999: 177–205; https://doi.org/10.1023/A:1008008021481.
22 Martens DE. Growth and Death of Animal Cells in Bioreactors (diss.). Wageningen University: Wageningen, the Netherlands, 1996; https://edepot.wur.nl/200254.
23 Ikonomou L, Schneider Y-J, Agathos SN. Insect Cell Culture for Industrial Production of Recombinant Proteins. Appl. Microbiol. Biotechnol. 62(1) 2003: 1–20; https://doi.org/10.1007/s00253-003-1223-9.
24 Tramper J, et al. Bubble-Column Design for Growth of Fragile Insect Cells. Bioprocess Eng. 3(1) 1988: 37–41; https://doi.org/10.1007/BF00372858.
25 Maranga L, et al. Scale-Up of Virus-Like Particles Production: Effects of Sparging, Agitation and Bioreactor Scale on Cell Growth, Infection Kinetics, and Productivity. J. Biotechnol. 107(1) 2004: 55–64; https://doi.org/10.1016/j.jbiotec.2003.09.012.
26 Vajrala SG, Murhammer DW. Effect of CO2 on Uninfected Sf-9 Cell Growth and Metabolism. Biotechnol. Prog. 32(2) 2016: 465–469; https://doi.org/10.1002/btpr.2229.
27 Wu H-C, Hu Y-C, Bentley WE. Tubular Bioreactor for Probing Baculovirus Infection and Protein Production. Meth. Mol. Biol. 1350, 2016: 461–467; https://doi.org/10.1007/978-1-4939-3043-2_23.
28 Green DW, Southard MZ. Perry’s Handbook of Chemical Engineering, 9th edition. McGraw Hill Education: New York, NY, 2019.
29 Camarasa E, et al. Influence of Coalescence Behaviour of the Liquid and of Gas Sparging on Hydrodynamics and Bubble Characteristics in a Bubble Column. Chem. Eng. Processing: Process Intensific. 38(4–6) 1999: 329–344; https://doi.org/10.1016/S0255-2701(99)00024-0.
30 Hughmark GA. Power Requirements and Interfacial Area in Gas–Liquid Turbine Agitated Systems. Ind. Eng. Chem. Process Des. Dev. 19(4) 1980: 641–646; https://doi.org/10.1021/i260076a023.
31 Chen Y. Simulation and Experimental Investigation of Power Consumption, Gas Dispersion, and Mass Transfer Coefficient in a Multi-Phase Stirred Bioreactor. Braz. J. Chem. Eng. 36(4) 2019: 1439–1451; https://doi.org/10.1590/0104-6632.20190364s20180450.
32 Nienow AW, et al. The Physical Characterisation of a Microscale Parallel Bioreactor Platform with an Industrial CHO Cell Line Expressing an IgG4. Biochem. Eng. J. 76, 2013: 25–36; https://dx.doi.org/10.1016/j.bej.2013.04.011.
33 Pamboukian MM, et al. Insect Cells Respiratory Activity in Bioreactor. Cytotechnology 57(1) 2008: 37–44; https://doi.org/10.1007/s10616-007-9118-8.
34 Paul EL, Atiemo-Obeng VA, Kresta SM, Eds. Handbook of Industrial Mixing: Science and Practice. Wiley: Hoboken, NJ, 2004.
35 Chisti Y. Mass Transfer. Kirk-Othmer Encyclopedia of Chemical Technology. Wiley Online Library, 2017; https://doi.org/10.1002/0471238961.1301191903080919.a01.pub3.
36 Iurashev D, et al. Scaling Strategy for Cell and Gene Therapy Bioreactors Based on Turbulent Parameters. Biotechnol. J. 19(1) 2024: e2300235; https://doi.org/10.1002/biot.202300235.
37 Haas R, Nielsen LK. A Physiological Product-Release Model for Baculovirus Infected Insect Cells. Biotechnol. Bioeng. 91(6) 2005: 768–772; https://doi.org/10.1002/bit.20565.
38 Joshi PRH, et al. Achieving High-Yield Production of Functional AAV5 Gene Delivery Vectors via Fed-Batch in an Insect Cell-One Baculovirus System. Mol. Ther. Meth. Clin. Dev. 13, 2019: 279–289; https://doi.org/10.1016/j.omtm.2019.02.003.
39 Moreno F, et al. Development of an Insect Cell-Based Adeno-Associated Virus Packaging Cell Line Employing Advanced Rep Gene Expression Control System. Mol. Ther. Meth. Clin. Dev. 27, 2022: 391–403; https://doi.org/10.1016/j.omtm.2022.10.015.
40 Sandro Q, et al. AAV Production Using Baculovirus Expression Vector System. Meth. Mol. Biol. 1937, 2019: 91–99; https://doi.org/10.1007/978-1-4939-9065-8_5.
41 Alavijeh MK, et al. Digitally Enabled Approaches for the Scale-Up of Mammalian Cell Bioreactors. Digit. Chem. Eng. 4, 2022: 100040; https://doi.org/10.1016/j.dche.2022.100040.
42 Harcum SW, et al. PID Controls: The Forgotten Bioprocess Parameters. Disc. Chem. Eng. 2(1) 2022; https://doi.org/10.1007/s43938-022-00008-z.
43 Heathman TRJ, et al. Agitation and Aeration of Stirred-Bioreactors for the Microcarrier Culture of Human Mesenchymal Stem Cells and Potential Implications for Large-Scale Bioprocess Development. Biochem. Eng. J. 136, 2018: 9–17; https://doi.org/10.1016/j.bej.2018.04.011.
44 Grein TA, et al. Aeration and Shear Stress Are Critical Process Parameters for the Production of Oncolytic Measles Virus. Front. Bioeng. Biotechnol. 7, 2019: 78; https://doi.org/10.3389/fbioe.2019.00078.
45 Iurashev D, et al. Scaling Strategy for Cell and Gene Therapy Bioreactors Based on Turbulent Parameters. Biotechnol. J. 19(1) 2024: e2300235; https://doi.org/10.1002/biot.202300235.
46 Walls PLL, et al. Quantifying the Potential for Bursting Bubbles To Damage Suspended Cells. Sci. Rep. 7(1) 2017: 15102; https://doi.org/10.1038/s41598-017-14531-5.
Corresponding author Tereza Pavlištová is a junior scientist in the Drug Substance Development department at uniQure B.V., Paasheuvelweg 25A, 1105BP, Amsterdam, the Netherlands; [email protected]. Maria João Alves Correia is a senior bioprocess technologist, Alejandro Molina Gil is a biotechnologist, Edina Ljubovic-Couteau is senior director of global drug substance development, Bas van de Waterbeemd is director of drug substance development, and Mathieu Streefland is vice president, all in the Global Process Development department of uniQure B.V. Now with Koppert Biological Systems, Hugo F. Cueto-Rojas was associate director and team lead of drug-substance development at uniQure at the time of writing.
You May Also Like