Assay Acceptance Criteria for Multiwell-Plate–Based Biological Potency AssaysAssay Acceptance Criteria for Multiwell-Plate–Based Biological Potency Assays
For most biopharmaceuticals, potency is assessed in a bioassay by comparing dose–response curves of the test material and a reference standard. As with all analytical techniques, such assays require criteria by which their execution can be judged objectively to be valid, regardless of whether the desired or expected result is obtained for the test sample.
PRODUCT FOCUS: BIOLOGICS
PROCESSFOCUS: R&D, QC
WHO SHOULD READ: PRODUCT AND PROCESS DEVELOPMENT, ANALYTICAL, QC
KEYWORDS: IMMUNOASSAYS, POTENCY ASSAYS, PRODUCT RELEASE, REFERENCE STANDARDS, CONTROL SAMPLES, SAMPLE ACCEPTANCE
LEVEL: ADVANCED
The purpose of this paper is to provide guidance on setting assay acceptance criteria (AAC) for potency assays based on multiwell plates. Multiple components of the overall assay system — from instruments to incubation media — need to be within defined limits to permit execution of a valid assay, so they are tested for suitability either before or during the assay. Because of the complexity of bioassay systems, not every relevant factor can be controlled. Thus, it is necessary to rely strongly on analysis of data produced by each individual assay to determine whether that assay was executed correctly. This paper discusses criteria that can be applied to the assay results and the importance of assay design in selecting useful acceptance criteria.
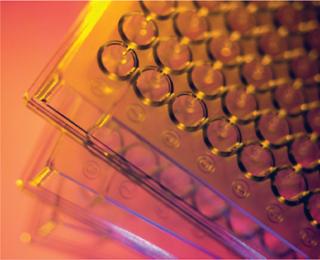
Scope
Why Multiwell-Plate–Based Assays Specifically? Analytic dilution assays using multiwell plates constitute the most common platform for measurement of biological activity by in vitro bioassay or measurement of immunoreactivity by immunoassay. The multiwell format provides a convenient means of handling the necessary number of doses and replicates, and it is supported by the availability of a wide range of plate types with a standardized footprint and supporting equipment and measurement systems. Multiwell-plate formats introduce specific artifacts to the measured responses. Thus, assay design — especially the positioning of individual samples within and between plates — is crucial to obtaining valid results and thus needs to be considered when setting acceptance criteria for an assay.
Abbreviations and Terms
4PL: four-parameter logistic nonlinear regression model
AAC: assay acceptance criteria
CV: coefficient of variation
ED50: the dose of a drug that induces a response that is 50% of the maximum response
PLA: commercial software package designed for the analysis of bioassay and immunoassay dose-response curves (Stegmann Systems GmbH)
R2: coefficient of determination
RSS: the residual sum of squares
SAC: sample acceptance criteria
Shewhart SPC: control chart named after Walter A. Shewhart
SPC: statistical process control
StatLIA: commercial software package designed for the analysis of bioassay and immunoassay dose-response curves (Brendan Technologies, Inc.)
Much of the following discussion is based on cell-based assays in 96-well plates, which reflects their wide use in potency testing. With some adaptation, however, many of the arguments can be applied to other assay systems and plate formats for plate-based potency assays.
Summary
This paper is restricted to discussion of tests applied to the responses of a bioassay system to reference standards, controls and test samples obtained during performance of a potency assay.
Assay acceptance criteria are based primarily on comparison of dose– response curves of control samples with a reference standard, all of which should be well characterized in the assay system.
At least one control sample should be known to behave similarly to the reference standard in the assay system. Both this control and the reference standard should be known to behave similarly to the expected behavior of test samples.
We propose the name assay control sample for the control material that behaves similarly to the reference standard and the expected behavior of the test samples.
The origin of the assay control sample should be as independent of the reference standard and test samples as is possible within the constraint that all behave similarly in the assay system.
For plate-based biological potency assays, we propose the following two separate sets of acceptance criteria: assay acceptance criteria (AAC) and sample acceptance criteria (SAC).
We propose a two-level, sequential assessment of acceptance criteria. First, AAC are assessed. Failure means that the entire plate is invalid. There is no processing of test sample data. Passing AAC allows processing of test sample data. Second, each test sample potency determination is then subjected to its own SAC. If it passes, then that test sample potency measurement is valid. If it fails, then that particular test sample potency quantification fails. Other test sample determinations on the same plate may still be valid.
Similarity of dose–response curves of reference standards and assay control samples is an essential AAC. Similarity of dose-response curves of the reference standard and test sample is an essential SAC.
AAC and SAC applied to an assay should be demonstrated to be useful in judging the validity of the assay. Both the criteria and the limits set on their values should be reviewed — and modified, if appropriate — as more assays are performed and further data are accumulated.
What Types of Assay? Considerations for setting AAC apply to a number of different assay types, including
functional assays (in which a biological response is measured)
biochemical assays (such as clotting-factor activity assays) considered as functional assays or as a separate class
binding assays (that measure binding of a ligand, receptor, cofactor, and so on), which may be potency assays, in which binding is the mode of action (MOA), or surrogate potency assays
immunoassays such as enzyme-linked immunosorbent assays (ELISAs) that measure binding of an antibody preparation (monoclonal or polyclonal) to one or more epitopes — distinct from functional assays in which binding of an antibody induces a functional biological response
hybrid assays (e.g., immunoassay-cofactor binding assays).
Many assays consist of multiple steps. For example, a functional assay may involve stimulation of cytokine secretion followed by immunoassay measurement of the secreted protein. AAC are generally set for the overall output, but may, in addition, be set for some individual steps. Potency assays for some types of products — e.g., advanced therapy medicinal products (ATMPs) — may require atypical assay designs and, consequently, atypical acceptance criteria.
AAC or System Suitability? Guidelines for testing a system to demonstrate its suitability for an analytical procedure have been developed primarily for physicochemical techniques. ICH Q2(R1) states that “a series of system suitability parameters (e.g., res
olution test) is established to ensure that the validity of the analytical procedure is maintained whenever used” and then provides specific examples for liquid and gas chromatography (1). USP chapter <621> on chromatography states, “To ascertain the effectiveness of the final operating system, it should be subjected to a suitability test prior to use. The essence of such a test is the concept that the electronics, the equipment, the specimens and the analytical operations constitute a single analytical system, which is amenable to an overall test of system function” (2). A useful definition, also from the field of liquid chromatography, is this: “System suitability is the checking of a system to ensure system performance before or during the analysis of unknowns” (3). So a system suitability test determines whether an analytical system is fit for use. In physicochemical analyses, making that decision may be possible before test samples have been committed to analysis.
As with physicochemical analyses, multiple components of the overall assay system need to be within defined limits to permit execution of a valid bioassay. For example, instruments, media, and environmental conditions are tested for suitability either before or during an assay. However, guidelines and examples designed for physicochemical techniques are not necessarily appropriate or sufficient for bioassays. Bioassays and (generally to a lesser extent) immunoassays tend to be susceptible to a greater number of factors than are most physicochemical analytical techniques. Some of those factors may be poorly controlled or not identified. For example, biological media may contain unidentified or undetected components that vary from batch to batch and affect the response of an assay system. Because such uncontrollable or unidentified sources of variability can cause assay-to-assay variability, it is necessary to rely strongly on analyzing data produced by each individual assay to determine whether that assay was executed correctly. Which tests of those data will be useful in determining the validity of the assay should be investigated during assay development and characterization.
The general term acceptance criteria has been defined to be “conditions which must be fulfilled before an operation, process or item, such as a piece of equipment, is considered to be satisfactory or to have been completed in a satisfactory way” (4).
Submission of Comments
The authors invite comments on this draft best-practice article from interested parties to assist in completion of a guidance on assay acceptance criteria for multiwell-plate–based biological potency assays. Send comments by email to the Biopharmaceutical Emerging Best Practices Association at [email protected]. Please start the subject line with “AAC.”
Comments should be received by 31 May 2014. All comments must be accompanied by a name, affiliation, and email address and will be given due consideration by the authors. Any comment submitted could be posted on the BEBPA website (www.bebpa.org/white-papers). Comments may be posted in their entirety, or extracts may be posted instead. In the latter case, it will be noted that only part of the comment is posted. If you would like your name and affiliation to be posted along with your comments, please state that BEBPA has your permission to do so.
What is the distinction between AAC and system suitability tests? The former are often considered to be a subclass of the latter. Both are set based on data acquired during assay development, characterization, and validation. System suitability may be checked either before or during the performance in which a test sample is assayed. The term assay acceptance criteria is generally used to describe conditions that must be met by data derived from an actual assay in which a test sample is assayed.
Because biological assay systems are more variable than physicochemical systems, it is generally necessary for more system testing to be run simultaneously with (rather than prior to) sample testing. For potency assays using multiwell plates, some tests are generally considered to measure system suitability and others are generally considered to assess assay acceptance criteria.
The important consideration for both system suitability tests and AAC is that they should be appropriate to the specific assay system, the precise purpose of the particular assay being performed, and the assay design. Classification of a particular test as a system suitability test or AAC may not be necessary or particularly helpful.
This paper is restricted to discussion of tests applied to data derived from the responses of test samples, controls, and reference standards obtained during performance of an assay intended to yield a potency value for a test sample.
Pharmacopeial Requirements: With pharmacopeial assays, all specified AAC will need to be met for those performed under relevant quality systems. Those criteria can provide useful guidance in setting criteria for similar assays and similar products. However, pharmacopeial assays generally specify fewer AAC than would be considered necessary for assays used for product release, so manufacturers would probably need to set additional AAC for release assays and develop appropriate criteria for novel assays and products.
Assay Acceptance and Sample Acceptance
For plate-based biological potency assays, we propose two separate sets of acceptance criteria: AAC and sample acceptance criteria (SAC). The former are based on responses of control samples and reference standards, and the latter are based on the responses of each separate test sample. Making this distinction permits the validity of an assay (or assay subsection) to be judged separately from that of each separate test sample. If an assay fails, then there is no processing of its test sample data. If analysis of data from one test sample fails the SAC, then that particular test sample potency quantification fails. Other test sample determinations on the same plate should be assessed separately and may still be valid.
Assay Acceptance: AAC are based on control samples and a reference standard. These are materials that are well characterized in the assay system and are independent of the test samples. At least one control sample should be known to behave similarly to the reference standard in this assay system, and both that control and the reference standard should be known to behave similarly to the expected behavior of the test samples. (See the “Similarity of Dose–Response Curves” section below.)
The control material that behaves similarly to the reference standard and test samples is often called the assay control, control material, or control sample. We propose adopting the term assay control sample. Other types of controls include those designed to exclude responses of a system that are not caused by specific actions of the test samples and those designed to demonstrate a positive response by a different mode of action. The former are often called negative controls and include materials such as excipients of the test sample or antibodies of a different specificity. Whereas the assay control sample and reference standard must be tested at multiple dilutions, other controls may be tested at one concentration or a few concentrations.
One feature of multiwell-plate–based assays that imposes constraints on assay design is plate-to-plate variability. Because of that, AAC should be applied to each plate independently, which means that dilution curves for an assay control sample and a reference standard should be run on every plate. If assay design constraints dictate that an exception be made to this rule, then it must have been clearly demonstrated durin
g assay characterization and validation that plate-to plate variability does not affect the assay’s validity.
AAC are based primarily on comparing dose–response curves of the assay control sample(s) with a reference standard, both of which should be well-characterized preparations. The origin of the assay control sample should be as independent of the reference standard and test samples as is possible within the constraint that all should behave similarly in the assay. Different lots of material from the same production method are commonly used. Two independent dilutions of the reference standard material (one serving as the reference and one as the assay control sample) are not sufficient; using two dilution series of the same material is testing only the dilution procedure and subsequent handling. In early assaydevelopment, however, an independent assay control sample may not be available, so using two samples of the reference standard could be the only option. In this case, the two samples should be processed as independently as possible, ideally starting from separate vials — and if possible, using alternative intermediate dilution schemes.
Ideally, the assay control sample and reference standard should be as similar as possible to the test samples in excipient formulation, concentration, aliquot volume, container, and so on. The assay control sample, reference standard, and test samples should be prepared and tested in exactly the same way. However, an initial step such as reconstitution of a lyophilized reference standard or dilution of a more concentrated solution may be necessary. Commonly, the reference standard may be presented in a formulation buffer different from that of the test samples to permit its storage as a frozen solution. It then requires dilution (concentration permitting) in the formulation buffer of the test samples to render it as similar as possible to the test samples. Similarly, the formulation of the assay control sample and other control samples should be brought as close as possible to that of the test samples.
The assay control sample must be compared with the reference standard and evaluated in terms of curve shape and potency. Assay control sample potency is evaluated against a statistically generated assay control chart. The concept of a control chart for the assay control sample is essentially simple: Multiple independent executions of an assay are performed in which the assay control sample is included as a test sample, with no preexisting expectations other than curve similarity to the associated reference standard. After a certain number of assay repetitions (e.g., n = 25 or n = 50), the arithmetic average and associated standard deviation (SD) of that value are determined for the assay control sample potency. A control chart acceptance criterion is then established using the mean assay control sample potency, with plus or minus some multiple of the SD defining the upper and lower limits of acceptability.
Although it is common practice to set statistical process control (SPC) limits at plus or minus three times the estimated SD (“Shewhart limits”), in many bioassay applications the estimated SD is based on only a few measurements and is thus a very uncertain estimate. An approach to setting SPC limits that takes this uncertainty into account is to use tolerance intervals (5). These intervals are wider when based on only a few measurements and (usually) become narrower as more and more data are acquired until, with an infinite amount of data, they become the same as the ±3 SD limits associated with typical Shewhart SPC charts.
In the article “Specification Setting: Setting Acceptance Criteria from Statistics of the Data” (6), upper and lower control limits (tolerance limits) are provided for as few as five assays (with a corresponding multiplier of ±10.75) to as many as 199 assays (with a corresponding multiplier of ±3.03). Using the table provided in this reference, one can establish a reasonable initial control chart with 25 assays (with a corresponding multiplier of ±4.05), and then revise those upper and lower control limits as the number of collected values for the assay control sample relative potency increases.
Sample Acceptance: SAC are tests applied separately to each test sample. Their passing or failing is independent of other test samples. Similarity of sample curve shape to that of the reference curve is a standard test. Other SAC (e.g., variability of replicates) are usually similar to those for reference and assay control samples, but some may be different. For example, with some assay systems (particularly those that may not use the whole dose–response curve), the SAC may allow for fewer doses to be used in the curve fit than for the reference standard or assay control sample. Limits may be set for the ED50 of test samples to ensure that the dose–response curve falls within the validated dose range.
The reportable value is defined in the testing protocol. It is typically the mean of a defined minimum number of assay results from valid independent assays, with “independent” being defined in the protocol. Most protocols specify that failure of the assay to meet AAC or of a sample to meet SAC more than a specified maximum number or percentage of times will trigger specified actions.
Assay Design
Use of multiwell plates introduces particular sources of potential artifacts in the measured responses of an assay. Wells in the corners, on the edges, and in the center of each plate have different environments, and the individual plates may be subject to slightly different conditions, any of which differences may affect responses. These effects are observed so commonly that the terms well effects, edge effects, and plate effects are widely used in the bioassay field. In many assays — particularly cell-based assays with long incubation times — the edge effects are so significant that often only the 60 center wells of each 96-well plate are used for measurements and the edge wells are filled with media or other solutions. Evaporation of medium from external wells, even in a humidified atmosphere, is a major contributor to edge well effects.
Recently developed 96-well plates with reservoir troughs around the edge can reduce the edge effect and, depending on the assay, may permit use of all 96 wells of each plate. During assay development, use of plate uniformity (the same dose applied to all wells, including the edge wells) can facilitate assay optimization by permitting assessment and possibly reduction or elimination of plate-positional effects, including edge anomalies. Nonrandom distribution of samples and doses — for example, placing all dilution curves for a particular sample in edge rows of the plates or reference standard in one plate and test sample in another — can introduce bias to measured relative potencies. Good assay design should reduce such bias. Completely random distribution of samples and doses is generally infeasible, so block or other structured designs can be used (7).
Reference standard and assay control sample dilution curves should be included on every plate to permit the AAC to be applied to each plate. In the past, some assay designs have included no assay control sample. In such cases, assay acceptance would be based solely on the reference standard dilution curve. That is a less rigorous test of assay validity, so this design should be avoided. In some designs, several plates are treated as one unit, which passes or fails the AAC as a single unit. Usually this is done to reduce the proportion of wells required for reference standard and assay control sample and to increase the number of wells available for test samples. Treating several plates as one unit is not recommended, however: Plate-to-plate variability can limit the use of this approach.
Within plates, replicate d
ilution series of the reference standard, assay control sample, and test samples should be placed in nonequivalent and nonsimilar positions (avoiding locating replicates of the dilution series all in edge wells, or all in center wells, in adjacent rows, all in the top of the plate, all in the bottom of the plate, and so on). Most assays are constrained by the number of wells and plates that can be used, so assay design is a compromise between the ideal and the feasible. The adequacy of a proposed design should be investigated during assay development and characterization, and the AAC should be selected and set accordingly.
Commonly Used AAC and SAC
Similarity of dose–response curves is an absolute requirement for determining relative potency. In current practice, some form of assessment is almost universally applied. It is recommended that at least one control sample known to behave similarly to both the reference standard and the expected behavior of test samples in the particular assay system (the assay control sample) be run as a full dose–response curve. Similarity of dose–response curves for the reference standard and assay control sample is an essential assay acceptance criterion, and similarity of dose–response curves of the reference standard and test sample is an essential sample acceptance criterion. Depending on the assay, whole or partial response curves (when doses do not permit an asymptote to be reached) and linear responses may be measured, and various combinations of criteria can be applied (see below). 4PL is commonly used for curve analysis. In-house programs and commercial software (e.g., PLA and StatLIA) are used. In some cases, the F-test is used initially to judge similarity and then, as historical data are acquired, it is replaced by equivalence testing. Against the recommendations of this paper, some assays do not include an assay control sample or other controls. In such cases, AAC are based on the reference standard dose–response curve or comparison of test sample and reference standard.
Curve Slope: Slope is an acceptance criterion applied in most assays, but in a few cases it is used for trending only. Most often, the ratio of slope is used, forming part of curve similarity testing. Limits on the ratio can be defined as confidence intervals or ranges (e.g., 0.80–1.25). In some cases, absolute values are defined for the slopes and used either in addition to the ratios or alone. Absolute values are based on validation or control chart data (e.g., mean ±3 SD from historical data). When setting limits of ±3 SD, care should be taken to use a sufficiently large data set. AAC limits can differ from those for SAC.
Lower asymptote is a useful AAC and SAC for some assays. Limits can be set as ratios, forming part of similarity testing, and as absolute values based on historical data. Lower asymptote values may be used only for trending.
Upper Asymptote: The same comments apply as for the lower asymptote, with the additional point that an upper limit may be placed on the value to account for instrument limitations, particularly when optical density (OD) read-outs are used. In the case of OD, when the read-out is the difference between OD at two wavelengths, care should be taken that neither exceeds the limit of the instrument.
Ratio of Upper Asymptote to Lower Asymptote: For some assays, an absolute value with limits can be assigned to this ratio, or limits can be set on the ratio between a reference and assay control sample or test sample of values for this ratio (ratio of ratios). The ratio of upper to lower asymptote can be useful for trending (e.g., indicating changes with cell passage number).
Inflection Point: The inflection point is not commonly used as an independent AAC or SAC, but proves useful in a few cases. In 4PL, this corresponds to the ED50.
ED50: The ED50 is an absolute value. ED50 is widely used for trending but not commonly used as an AAC or SAC. The ratio of ED50 of reference to ED50 of assay control sample or test sample is sometimes used to assess potency.
Goodness of Fit: Goodness of fit is an essential AAC and SAC and is almost universally applied. R2 ≤ 0.95 to R2 ≤ 0.98. Testing R2 is relatively simple, but R2 may not provide a very sensitive indicator of goodness of fit. During assay development, it can be useful for selecting the most appropriate model to plot residuals against dose to reveal whether there is a random or a systematic deviation from the model. Residual sum of squares (RSS) and chi squared (χ2) are also used, the latter primarily for quantal assays. The most appropriate model and test depend on the individual assay and should, as with all acceptance criteria, be checked during routine use.
Potency of Control: With the exception of a few unusual assays, an assay control sample (run as full dose–response curve) should be included in every assay — usually on every plate. At present, some assays do not include any control samples and base the AAC on the reference standard alone or in comparison with the test sample. Other assays include a positive control at only one dose level or a few dose levels. Limited space on assay plates is one reason for the latter case. Limits ranging from 70–130% to 90–110% are typical, with 80–125% being very common. Limits are often set based on historical data (e.g., mean ± 3 SD). When setting limits of ±3 SD, care should be taken to use a sufficiently large data set. And care should be taken to define assay blanks and negative controls. Blanks usually refer to the absence of the active pharmaceutical ingredient (API), corresponding to the addition of a sample of the formulation buffer. Negative controls usually consist of relevant substances without a specific action in the particular assay (e.g., in the assay of a therapeutic antibody, a negative control might be antibody without specific binding to the ligand used in the assay).
Ratio of Values for Different Control Samples: This ratio is not commonly used. Use of more than one positive control is not common, but the ratio of high to medium or high to low positive controls, for example, can be useful AAC. The value of the ratio of positive to negative control responses will vary greatly with changes in response of the negative control if the negative control is very small.
Minimum Number of Doses Used in Curve Fitting: Usually set as an AAC and SAC, the value depends on the particular assay and whether it is a linear or full curve fit. Usually all doses are required, but in some cases exclusion of one or more points is permitted (see “Maximum Number of Statistical Outliers Excluded” section below). Depending on assay design, this generally results in a minimum of 6–10 doses, with eight being very common. For some assays (e.g., those in which wide ranges of test sample potencies may be encountered) a smaller number of doses, specified as being consecutive, may be set as a SAC.
Minimum Number of Doses in “Linear” Part of Dose– Response Curve: Usually set as an AAC and SAC, both for linear and full curve fits; values generally range from three to six, with three and four being most common.
Minimum Number of Doses in Upper and/or Lower Asymptote: This criterion is used in some assays with the most common value being two. This criterion is sometimes used during development and subsequently discarded.
Minimum Dose Range Used in Curve Fit: Sometimes set explicitly as an assay acceptance and sample acceptance criterion, this commonly specifies that a minimum dose range (e.g., 50–150%) should lie within the linear range. In many assays, the minimum dose range is set implicitly as an acceptance criterion because the protocol sp
ecifies doses tested and the minimum number of doses used in curve fitting.
Maximum Number of Statistical Outliers Excluded: For many assays, a maximum number of statistical outliers that can be excluded is set as an assay acceptance and sample acceptance criterion. This can be defined as the number of doses (all replicates) or individual points that can be excluded per curve, or the number of replicates per dose, or a combination of these. The statistical test used to identify an outlier should be defined and have been shown to be suitable for the assay. Limits on the number of permitted exclusions depend strongly on the assay. Typical examples are one dose or one point per eight-point curve or per sample. Many assays allow no exclusion of outliers; others allow exclusion only if an anomaly can be attributed to experimental error. To prevent bias, any point suspected of being subject to experimental error normally should be excluded before its value is determined. However, it is recognized that experimental error may sometimes be identified only after a point is observed to be anomalous.
Variability of Replicates: Assessing the variability of replicates is essential, with limits normally set as AAC and SAC. Replicates commonly consist of the following:
Replicate wells containing the same solution (aliquots of the same dilution point in a single dilution series)
Replicate dilution series from a common starting solution
Replicate aliquots, vials, ampules, and so on.
The data may be analyzed from one multiwell plate or across several plates.
When variability of replicates is quoted, what is replicated must be defined exactly. In the first case above, the variability measures the errors in pipetting solution into the wells and errors introduced in subsequent steps. It does not assess errors in preparation of the starting solution or the dilution series. For measuring relative potency, ideally the handling of each sample should be the same, and each replicate should capture independently as many steps as possible from the handling process.
Variability depends on the assay. It is commonly expressed as%CV. For enzyme-linked immunosorbent assays (ELISAs) and cell-based potency assays for products such as cytokines or MAbs, AAC and SAC are generally set at%CV 10–30%. For vaccine assays, in which dilution series steps of log10 may be used, considerably broader limits may be acceptable depending on the protocol and requirements of a given assay. An alternative option is to set a SAC as the relative 95% confidence interval around the mean value for test sample relative potency (e.g., at 80–125% or 75–133%).
The requirements for the limits on assay variability are often subject to requirements for the precision of the assay result. If greater precision is required, one approach can be to modify the assay protocol to include more replicates.
Although variability between replicate wells of one dose (measured by CV) is the most widely used assessment of replicate variability, other assessments may be used (e.g., variability between replicate potency determinations from single dose curves) depending on the assay design. Variability of replicates is one of the most important tools for trending.
Selection of assay and sample acceptance criteria is inextricably linked to assay design. For example, if replicate curves are made on a single plate, then acceptance criteria might include limits on the coefficient of variation (CV) for the slopes of the replicate curves. If separate potency determinations are made from each plate in a multiplate assay, then acceptance criteria limits might be set on the CV of potency determinations. Commonly used acceptance criteria and some typical assigned values are listed in the “Commonly Used AAC and SAC” boxes.
Monitoring and Trending
Values observed for assay (and sample) acceptance criteria can be used for assay (and sample) monitoring and trending. For the simplest case of assay monitoring, visual inspection of run charts (trend charts) displaying observed data in a time sequence can reveal aberrant results and indicate whether an assay is stable or drifting. Comparison with the results of system suitability tests (e.g., cell density before harvest for an assay or degree of cell confluence before dosing) and records of critical reagents and equipment can help reveal the cause of drift or aberrant results.
In addition to assay and sample acceptance criteria, some parameters are reported “for information only.” Those parameters do not determine the acceptance or failure of an assay orsample, but they can be useful in monitoring and trending. Monitoring and trending of as wide a range as feasible of assay response characteristics is useful, particularly in the early stages of assay development and use. As data accumulate over a large number of assays, incorporating variability in operating conditions (such as reagent batches and operators), it can become evident which characteristics may be useful for continued monitoring and trending and which should be adopted or retained as acceptance criteria. Inclusion of an assay response characteristic in monitoring or trending should not carry an automatic expectation that it should be adopted as an acceptance criterion and have limits set. Such an expectation can discourage the investigation of response characteristics and eventual selection of optimum sets of acceptance criteria.
When statistical analysis is applied to observed values, a statistical process control (SPC) chart can be obtained, permitting an objective analysis of the variation in assay performance and allowing limits to be set to indicate when action must be taken to prevent an assay drifting into failure. “The use of statistical control charts to map the ongoing performance and stability of reference material during routine assays can be a useful quality control tool allowing for early detection of adverse trends” (8) and “SPC charts are a powerful tool for showing auditors the continual validation of an assay” (9).
Evolution of Acceptance Criteria During Development
Assay and sample acceptance criteria (and their assigned values) will change during assay and product development processes as a result of improvement in assay performance, accumulation of more data on that performance, and stricter requirements at later stages of product development. Even when an established assay platform is applied to an additional product, it should be expected that there will be a development process for establishing assay and sample acceptance criteria.
During assay development and on accumulation of performance data, it would normally be expected that limits on some criteria would be tightened. However, it may become apparent that initial data collected over a short period of time did not reflect the full variation in assay conditions (e.g., variation in reagent batches) that will be encountered over a longer period. The criteria may therefore be initially set too tightly and subsequently need to be widened. Additional criteria may be included later on. Conversely, with accumulation of data, it may become evident that some criteria initially set do not reflect the assay’s validity. Those criteria should be removed as they do not contribute to assessing assay validity, and their inclusion could result in rejection of assays that are fit for purpose. Their values can be recorded for the purposes of monitoring and trending.
Acceptance Criteria
Absolute and Relative Values: A potency assay is comparative, measuring the potency of a test sample or control sample relative to that of a reference standard. A comparative assay is based on the assumption that a factor that affects the response of a system to a test sample should affect the response of that system to a standard sample equally. Therefore, absol
ute values for characteristics of a response curve should not be critical. In practice, however, most assay systems function adequately over only a limited range of conditions reflected by a limited range of acceptable values for some of the dose–response curve characteristics. An unusually high or low value for the ED50, for example, can indicate that an assay system is not behaving as usual.
Similarity of Dose–Response Curves: The response of a bioassay system to a sample can be affected by a variety of external factors, some of which may not be controlled, so potency measurement cannot be an absolute value. Bioassays are comparative, with the biological activity of a test material measured relative to that of a reference preparation (10). If two preparations are sufficiently similar, then their responses should be affected equally by any variation in the system, and relative potency should remain constant. It is a fundamental requirement for obtaining a valid relative potency that the reference standard and test sample must behave similarly in an assay system and hence their dose–response curves must have the same mathematical form. Any displacement between those curves along the log-concentration axis must be constant at all responses. This constant displacement is used to estimate relative potency. Nonsimilarity of two preparations may lead to dose–response curves of different mathematical forms with variation in the magnitude of displacement between the curves. In this case, any attempted measurement of relative potency would vary depending on the response level at which it was measured.
Similarity of dose response is thus an essential assay acceptance criterion. To determine whether a reference standard and test sample demonstrate the same dose–response relationship in a given bioassay, it is necessary to measure the response of each at several doses spread over an appropriate range. Assessing the similarity of dose–response curves depends on statistical analysis of the data. For some pharmacopeial assays, the method of analysis may be specified precisely. In many cases, however, only general guidance can be obtained from pharmacopeial sources. It is beyond the scope of this paper to discuss the relative merits of different statistical methods, such as the F-test and equivalence testing, for assessing similarity in various circumstances. Further information can be found in the literature (11,12,13,14,15).
Consultation with a statistician who is experienced specifically in analysis of bioassay data is strongly recommended during assay development and validation to assist in establishing methods of data analysis appropriate to a particular assay and setting appropriate AAC.
For many well-characterized bioassays, a mathematical transformation of the response is selected to give a linear relation (over a range of doses) with log dose. This is the parallel-line assay and, in this case, dose–response curve similarity isreferred to as parallelism. Even when parallel-line analysis is used, it may be appropriate to use asymptotic values as additional assay acceptance criteria.
With the increasing availability of software packages, four-parameter logistic (4PL) curve fitting is widely used. The four parameters are minimum asymptote (A), Hill slope (curve steepness, B), inflection point (C) and maximum asymptote (D). Each of these parameters may provide an assay acceptance criterion for determining similarity of the dose–response curves of test samples and reference standards and/or as an absolute value. 5PL fits are used less commonly but can provide a better fit for dose–response curves that are not symmetrical around the inflection point.
The “Commonly Used AAC and SAC” boxes list commonly used AAC and SAC, including comments on their utility and some typical values. AAC are applied to reference standard and control samples, whereas SAC are applied to each individual test sample. The list incorporates information provided by delegates at the 2013 BEBPA bioassay conference (16).
Common Practices That Cause Problems: Certain common practices in setting acceptance criteria are the cause of frequent problems. One such practice is acceptance being made too dependent on an individual data point. A protocol illustrating this point involves standard, assay control sample and test samples tested in duplicate dose-response curves on a plate. Upper asymptotes are determined as the mean of duplicate responses at maximum dose with a maximum acceptable percentage difference between upper asymptotes on one plate. The reportable value is the mean of the potencies determined on two replicate plates, with a maximum acceptable percentage difference between the two potencies. One aberrant well at the maximum dose of the assay control sample on one plate results in the upper asymptote of the assay control sample being aberrant compared with the reference standard so the plate is rejected. No reportable value is obtained because of one aberrant well out of 120.
Another common cause of problems is failure to ensure that each of the acceptance criteria is valuable in judging the validity of the assay as established during the assay characterization and continued monitoring. Inclusion of unnecessary criteria may result in the rejection of assays that are in fact fit for purpose. For example, in some systems, ED50 may vary widely between assays which are fit for purpose, so setting tight limits on an absolute value for ED50 could result in pointless rejection of an assay.
About the Author
Author Details
Corresponding author C. Jane Robinson is a principal scientist at the National Institute for Biological Standards and Control in South Mimms, UK ([email protected]). Michael Sadick is senior manager of biopharmaceutical characterization at Catalent Pharma Solutions in Kansas City, MO ([email protected]). Stanley N. Deming is president of Statistical Designs in Houston, TX ([email protected]). Sian Estdale is a principal scientist in large-molecule biopharm CMC at Covance Laboratories Limited in Harrogate, UK ([email protected]). Svetlana Bergelson is director of analytical development at Biogen Idec in Cambridge, MA ([email protected]). And Laureen Little is principal consultant at Quality Services in Seattle, WA; [email protected]
REFERENCES
1.) ICH Q2(R1).
2.) Chapter <621> ChromatographyUSP29–NF24, US Pharmacopeial Convention, Rockville.
3.) Shabir, GA 2003. Validation of High-Performance Liquid Chromatography Methods for Pharmaceutical Analysis: Understanding the Differences and Similarities Between Validation Requirements of the US Food and Drug Administration, the US Pharmacopeia, and the International Conference on Harmonization, J. Chromatography A 987:57-66.
4.) United Nations Office on Drugs and Crime 2009. Guidance for the Validation of Analytical Methodology and Calibration of Equipment Used for Testing of Illicit Drugs in Seized Materials and Biological Specimens, United Nations, New York.
5.) Hahn, GJ, and WQ. Meeker
. 1981.Statistical Intervals: A Guide for Practitioners, Wiley, New York.
6.) Orchard, T 2006. Specification Setting: Setting Acceptance Criteria from Statistics of the Data. BioPharm Int. www.biopharminternational.com/biopharm/Article/Specification-Setting-Setting-Acceptance-Criteria-/ArticleStandard/Article/detail/390955. 19:22-29.
7.) Lansky, D 2002. Strip-Plot Designs, Mixed Models, and Comparisons Between Linear and Nonlinear Models for Microtitre Plate Bioassays in the Design and Analysis of Potency Assays. Dev. Biol. 107:11-23.
8.) CBER 2011. Guidance for Industry: Potency Tests for Cellular and Gene Therapy Products (draft guidance), US Food and Drug Administration, Rockville.
9.) Deming, S 2008. Trending, Statistical Process Control and Continual Validation. NEPDA Newsletter www.pda.org/Chapters/North-America-cont/New-England/Chapter-News/January-2008-Newsletter.aspx. 3:1-3.
10.) Lightbown, JW 1961. Biological Standardization and the Analyst: A Review. J. Soc. Anal. Chem. 86:216-230.
11.) Chapter 5.3: Statistical Analysis of Results of Biological Assays and TestsEuropean Pharmacopoeia 8, European Directorate for the Quality of Medicines and Health Care, Strasbourg.
12.) Chapter <1034> Analysis of Biological AssaysUSP36–NF31, US Pharmacopeial Convention, Rockville.
13.) Hauck, WW. 2005. Assessing Parallelism Prior to Determining Relative Potency, PDA. J. Pharmaceut. Sci. Technol. 59:127-137.
14.) Callahan, JD, and NC Sajjadi. 2003. Testing the Null Hypothesis for a Specified Difference: The Right Way to Test for Parallelism. BioProcessing J.
15.) Plikaytis, BD. 1994. Determination of Parallelism and Nonparallelism in Bioassay Dilution Curves. J. Clin. Microbiol. 32:2441-2447.
16.) 2013..
You May Also Like