The classic F-test for nonparallelism is widely used for bioassays with linear log dose-response lines to assess parallelism, or, more correctly, to examine the strength of evidence against a null hypothesis that the two lines are parallel. Alternative methods for assessing parallelism have been proposed, but their suitability for any particular case needs to be carefully considered. Here we examine some advantages and disadvantages of the different approaches.
PRODUCT FOCUS: ALL BIOLOGICALS
PROCESS FOCUS: QA/QC
WHO SHOULD READ: QA/QC, ASSAY DEVELOPMENT, AND ANALYTICAL PERSONNEL
KEYWORDS: PARALLELISM, BIOASSAYS, STATISTICAL ANALYSIS, PRODUCT TESTING
LEVEL: INTERMEDIATE
Why Bioassays Are Necessary: For most biological therapeutic products and vaccines, a bioassay for potency measurement is a required part of the specifications for batch release. Potency is defined in ICH Q6B as “the measure of the biological activity using a suitably quantitative biological assay (also called potency assay or bioassay)” and biological activity as “the specific ability or capacity of the product to achieve a defined biological effect”(1).
Biological activity depends on the integrity of certain features of a molecular structure, often including higher-order structure which cannot be assessed by physicochemical methods.
In many cases, a bioassay is the only means to assess these aspects of molecular structure and predict the potency of a preparation. A bioassay that gives a measurable dose–response relationship (based on a product attribute linked to biological properties relevant to its clinical action) is therefore essential for quality control and calibration. In addition to specifications for batch release, bioassays play an important role in stability, comparability, and equivalence studies.
Relative Potency
Bioassay systems are complex and tend to be sensitive to a greater variety of factors than are most physicochemical techniques. Some factors can be controlled, but some cannot, and some may not be identified. Variation in these factors affects the response of a bioassay system to a test product, so its potency measurement is not an absolute value. Bioassays are therefore comparative, with the biological activity of a test material measured relative to that of a reference preparation (2). If the reference preparation is very similar to the test product, then their measured biological dose–response relationships should be affected equally by any variation in the system. Relative potency should therefore remain constant even though the measured response may vary among assays.
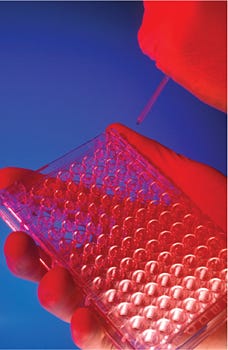
Figure 1:
WWW.PHOTOS.COM
A fundamental assumption essential to the concept of relative potency is that the two biological preparations compared (the reference standard and the test product) must behave similarly in the assay system. One preparation must behave as though it is a dilution of the other in a completely inert diluent. As a consequence, their dose–response curves will have the same mathematical form. Any displacement between the curves along the concentration axis is constant and is a measure of relative potency (Figure 1). Nonsimilarity of two preparations may lead to dose–response curves of different mathematical form with variation in the amplitude of that displacement. So any attempted measurement of relative potency would vary depending on the concentration at which it was measured.
Figure 1: ()
To determine whether two preparations demonstrate the same dose–response relationship in a bioassay, it is necessary to measure the response of each one at several doses spread over an appropriate range. Measuring the response of a preparation at a single dose does not permit comparison of dose–response curves. Assessing the similarity of dose-response curves depends on statistical analysis of the resulting data.
Parallel Line Assay and the F-Test
For many established or well characterized bioassays (e.g., pharmacopoeial assays), a mathematical transformation of the response is selected to give a linear relation (over a sufficiently wide range of doses) with log dose. This is the so-called “parallel line” assay. One-way analysis of variance is widely used to compare the means of differently treated groups and is the statistical method customarily used for analysis of such an assay. For bioassays, each preparation at each dose level constitutes a “treatment” of the bioassay system. The sum of squares between treatments is subdivided to give tests for overall difference between preparations, linearity of the transformed dose-response lines, and parallelism of reference and test preparations.
KEY POINTS
Functional similarity of preparations is a fundamental requirement for determining a valid relative potency. For bioassays with linear log dose–response relationships, this leads to the requirement that the lines for preparations compared are parallel. Statistically significant nonparallelism can compromise the acceptability of a bioassay even if it is believed to be of little or no practical significance.
The classic test for parallelism is the F-test, which essentially compares the difference in slopes of dose-response lines with the random variation of individual responses. Even in assays that do not meet all statistical assumptions required for valid use of the F-test, it generally provides a useful starting point for assessing the similarity of log dose-response lines. The utility of alternative statistical tests in specific situations is recognized in pharmacopoeia.
Often, during assay development, reduction of random variation improves assay precision. Previously obscured differences in slopes of dose–response lines (whether due to systematic variation in an assay or true dissimilarity in the preparations) may then become apparent.
One proposed alternative to the F-test that is currently being widely discussed permits what is described as an “acceptable” degree of nonparallelism. Such a method would need to be used with caution: Appropriate definition of the acceptable limits of nonparallelism in any individual case is crucial and requires an understanding of the origin of that nonparallelism and its implications in clinical or other applications and requires historical empirical data for each specific assay.
The fundamental requirement for similarity of dose–response relationships cannot be ignored, and the most appropriate method for assessing similarity in the context of the nature and design of specific bioassays must be carefully considered.
Such analysis provides classic tests for parallelism, linearity, and differences between preparations based on the F-test and is the method widely adopted by pharmacopoeias. Essentially, it assesses nonparallelism by comparing the difference in slopes of two dose–response lines with the random variation of their individual responses — or noise.
The F-test is a test for the null hypothesis that the slopes of a reference and a test preparation are equal, with the alternative hypothesis being that their slopes are not equal. That null hypothesis cannot be shown to be true. Thus, it cannot be concluded that the slopes are equal. It can be concluded, however, that the two parameters do not differ by a greater amount than the difference detectable using available data.
The power of the test to detect differences depends both on the magnitude of the difference to be detected and the precision of the available data. That is, to accept the null hypothesis does not show that it is true, but rather shows only that for the observed data with their observed variability, the observed difference is not so large as to exclude the null hypothesis. In other words, the null hypothesis has not been shown to be false, and it has not been proved that the curves are nonparallel and the preparations dissimilar, so there remains the possibility that those curves may be parallel and the preparations similar. To reject the null hypothesis shows (at the probability level of the test) that it is not true: It shows that the curves are not parallel so the preparations are not similar.
A mistake sometimes encountered is the interpretation of accepting the null hypothesis as showing that the two slopes are equal. It must be emphasized that this classic test cannot be taken to prove parallelism. However, its power can be determined, and the difference that would have to be detected to establish nonparallelism (at the specified probability level) can be calculated. Unless otherwise indicated, we use the word difference in its general sense here — meaning “not the same” or “not equal” — without the nature of the difference being specified.
As mentioned, bioassays are subject to many sources of variability. The response of an in vitro bioassay may be affected by differences in, e.g., batches of media, age of cell stocks, speed of reagent additions, or shear forces during mixing. Control of some variables commonly improves during assay development, and improvement may continue even after the assay is regarded as characterized or established. Reducing the sources of random variation improves intraassay (repeatability) and interassay (intermediate precision and reproducibility) precision. Some nonrandom (e.g., systematic) sources of variation may remain, however, and improvement in precision can be accompanied by an increase in nonparallelism as judged by the F-test. This is because differences in the slopes of dose–response lines (whether caused by systematic sources of variation or true dissimilarity in the preparations) that were previously obscured become apparent as random variation (“noise”) of the data decreases.
Nonparallelism
Detecting nonparallelism can have serious consequences. For established assays, detection of statistically significant nonparallelism between dose–response lines for test and reference materials may lead to rejection of a sample and failure of a batch — or a requirement to retest. Absence of statistically significant nonparallelism between dose–response lines for reference and/or control samples often forms part of the assay acceptance (system suitability) criteria. So when nonparallelism is detected, an assay may need to be rejected.
The nature of bioassay analysis and the susceptibility of bioassays to so many variables (some of which may not be controlled or controllable) mean a certain portion of assays and/or samples may give incorrect results — including, e.g., a false result demonstrating nonparallelism. In recognition of this fact, protocols are developed to define the actions to be taken on failure of sample or assay. Such protocols may allow for retesting of a sample or retention of an assay if only a limited portion of a set of acceptance criteria fails. Development of such protocols is based on many factors, usually including the history of an assay’s performance.
If reduction of random variation in an assay system leads to emergence of previously obscured nonparallelism, more test samples or assays may be rejected or more retesting may be required. For this reason, a criticism sometimes made of the F-test is that an improvement in assay precision can be punished by the emergence of nonparallelism. In some cases, such statistically significant nonparallelism is believed to be of little or no practical significance. This is not a new problem (2) and has led to proposals for using alternative statistical tests. Some propose allowing an “acceptable” degree of nonparallelism (3,4,5). One recent suggestion is based on an equivalence-testing approach that proposes to test a different null hypothesis (rather than the classic hypothesis of equal slopes), namely that two slopes may differ by some specified amount but that this difference is negligible and that they may considered equivalent. Although this type of approach may be possible in some circumstances, it requires careful consideration of several factors in the context of each specific case as we discuss later on.
Origin of Nonparallelism: Parallelism is a fundamental and essential assumption for validity of relative potency estimation. Demonstration of nonparallelism shows functional dissimilarity of the two preparations compared and thus invalidates an estimate of relative potency. It is impossible to conclude that any level of nonparallelism is trivial with respect to potential clinical consequences without understanding the origin of that nonparalellism.
A simplistic analogy from physicochemical assays might be the detection of a small difference (a fraction of a percent) in molecular weights of two batches of a biopharmaceutical, a difference that may be apparent only on improvement or change of the analytical technique. The difference could be indicative of an amino acid mutation, and the change of one amino acid may be without clinical consequence — or it may fundamentally change the biological properties of a molecule (6). So the molecular weight difference cannot be dismissed as trivial simply on the basis of its magnitude.
A situation that can arise is that of early batches of product that prove satisfactory in the clinic and are tested by an imprecise assay with no apparent nonparallelism between test and reference — but later batches show non-parallelism when tested in a more precise assay. Rather than simply attributing this emerging nonparallelism to the improved precision of the assay, archived samples of the early batches should be tested in that improved assay if possible to demonstrate comparability of the clinical trial and later batches of product. It may then prove necessary to reconsider the suitability of the reference standard.
If statistically significant nonparallelism is evident for preparations known to satisfy the assumption of similarity — for example, aliquots of the same sample — then assay design and procedures must be examined for violations of the assumptions underlying the statistical analysis. In such cases, a modified assay design or more suitable analysis may be appropriate. This is recognized in most pharmacopoeial monographs by the allowance of alternative justified and validated statistical methods.
Bioassay Design and Assumptions Underlying Statistical Analysis
When considering the use of bioassays, it is important to distinguish between the fundamental validity of an assay and the validity of the mathematical and statistical treatment of the resulting data. If a fundamental assumption is not true for a given assay, then data obtained cannot lead to a correct result no matter what arithmetical processes are applied. If, however, one or more of the assumptions for statistical validity is untrue, then it may be possible to amend the assay design and/or the method of computation. For any final statement of potency and its limits to be valid, both fundamental and statistical validity are required (7).
A number of data-related assumptions underlie all statistical methods. The design of a bioassay must meet these assumptions as closely as possible or try to compensate for factors that may violate them. One assumption on which the F-test is based is that experimental units are an independent random selection from a defined population and that responses are determined completely by dose. In reality, this is rarely the case. As an example, consider a common assay design in which serial dilutions of samples are loaded onto 96-well cell culture plates. Wells in the corners, on the edges, and in the center of one plate differ in their environments, and separate plates may be subject to slightly different conditions, any of which may affect responses in those units (wells and plates).
Figure 2 shows an example assay readout from cells cultured in 96-well plates in which each well was treated identically. For visual impact, the optical density readout is ranked into four grades based on the magnitude of response. It is obvious that the cells in each well, although treated identically, do not show an identical response. The distribution of responses within a plate is not random, and the two plates show a different distribution pattern. Although a good assay design would seek to reduce this variability and compensate for those factors that could not be eliminated, it is not easy to achieve such an ideal in practice. For some factors, block or other structured designs can be considered (8), but they are not always practicable or feasible.
Figure 2: ()
In many 96-well plate assays, samples and doses are not distributed completely randomly. Thus, as can be seen from Figure 2, bias can be introduced to the measured dose–response relationship. A completely random distribution of samples, on the other hand, might lead to a greater delay between dosing the first and last wells, thus introducing yet another factor that can affect the measured response.
Serial dilution of samples is a common practice (especially in 96-well plate assays) that is rapid and logistically simple, thus reducing operator error, and economical in its use of sample material. However, it can lead to an error being propagated systematically through the dose series of a sample, causing nonparallelism of dose–response curves because the responses at any dilution are not independent, but rather depend on the preceding dilutions. Figure 3 shows the divergence of three nominally identical dilution curves of the same material. Such divergence may be the result of propagated dilution errors causing nonparallel dose–response curves. Alternatively, there might be row effects that become apparent only at higher response levels, or there could be some other unrecognized source of bias.
Figure 3: ()
Various features of experimental design can reduce such bias or permit an assessment of its effect. One simple method of direct assessment is treatment of identical preparations as independent samples and measuring the nonparallelism of their dose–response lines (and their relative potency, which should equal 1). Blinding operators to the identity of the samples removes further possible sources of bias so coded or hidden replicates are included in some assay designs.
In some bioassays, known differences between reference standard and test preparation in excipient composition or slight molecular modifications of the biological material affect the response, leading to a degree of nonparallelism. Such violations of the fundamental principle of functional similarity do not constitute a statistical problem, so there should be no attempt to apply a statistical adjustment to disguise them. When nonparallelism arises because of the formulation, it may be possible to adjust assay conditions to obtain parallel dose–response curves — by, for example, adding components to the test or reference solutions that reduce differences in their excipient composition. An example of dealing with nonparallelism arising because of dissimilarity of the materials is seen in the measurement of serum antibody concentrations in a range of samples for which results depend on the dose range of the sample, and different assay systems may give different results (5). A possible violation of the fundamental assumption of parallelism is recognized, and the extent of such violation may even be quantified. For such a situation, sample dilutions covering a broad dose and response range can be used to derive a “representative” value of relative potency (5).
If no such suitable adjustment of the assay system is possible, then the appropriate action — which would have to be agreed upon with a regulatory authority and supported by clinical or other data — may be to accept the nonparallelism until, for example, more suitable reference standards or assay systems can be developed (9).
Statistical Alternatives to the F-test
Specific experimental situations may require particular statistical approaches. This was recognized by R.A. Fisher in discussing the validity of estimates of error used in significance tests (10). He noted the problem that “standardized methods of statistical analysis have been taken over ready-made from a mathematical theory, into which questions of experimental detail do not explicitly enter”. It is frequently the case that estimates of residual error calculated for individual biological assays do not satisfy the statistical assumptions required for a classic F-test. This issue is explicitly recognized in the European Pharmacopoeia (EP), which suggests ways to overcome the problem. Alternative approaches should be adopted when they are appropriate and validated for a specific experimental situation.
Various alternative approaches have been suggested for specific situations. Modifications of the acceptance criteria for classic statistical tests form the basis for some. For example, Story et al. propose a multiplication factor for the F value and present empirical data for it in the context of a particular assay (11). In some circumstances, an estimate of the residual error based on historical or validation data may be used, as suggested by the EP. This requires empirical data and ongoing validation of the estimate used. Other alternative methods are based on consideration of the dilution profile of a test sample, and acceptability of potency estimates is assessed using the consistency and magnitude of changes in the estimates of potency for a dilution series of the test sample (5, 12).
An approach recently suggested is based on equivalence testing (3, 4). It proposes that the hypothesis of the classic test is “flawed,” and bases its alternative approach on showing that two lines are “sufficiently parallel.” The problem Hauck et al. identify is that “perfectly acceptable assay results may fail due to good precision” and that “obviously faulty assay results may pass due to poor precision” as illustrated in Figure 3 of Reference 3,. Limits are thus set for the acceptable magnitude of the difference in slopes and for the precision associated with that difference. Although the F-test and this equivalence test lead to the same conclusion in many cases (Figure 4, example A95), they can in certain cases lead to different conclusions concerning parallelism and hence functional similarity of biological preparations (Figure 4, examples B95 and B99).
Figure 4: ()
Under the equivalence-testing approach, it is proposed that two lines with slopes that differ significantly from one another but for which the difference is “statistically less” than some specified value should be described as “equivalent” (Figure 4, example B95). This distinguishes the equivalence approach from the classic F-test approach. The approach has proved useful in some circumstances. For example, in comparisons of two different clinical treatments or drugs, it may be required that a statistical test have the power to detect differences of a specified (clinically important) magnitude, and it may be further considered that the cost of changing treatments is not justified unless the difference in treatments is “sufficiently large.” The definitions of large or clinically important differences must be defined in the context of a particular biological or medical situation. A crucial question for this approach is then how the criterion of sufficiently parallel might be defined for a biological assay — and more critically, what the implications might be of any proven deviations from parallelism even though such differences fall within some specified interval.
Again, the word difference is used in its general sense here. However, to apply the equivalence-testing approach, it is necessary to select and define some measure of the difference in slopes (e.g., the absolute numerical difference of slopes or the deviation of the ratio of slopes from a value of 1.0). It is then necessary to specify how a confidence interval for the selected measure of difference can be calculated.
A situation where obviously faulty assay results may pass due to poor precision is unacceptable.It must be noted that low assay precision is an issue distinct from assay validity, however, and possible consequences are not limited to the failure to detect nonparallelism. Various criteria will determine the level of assay precision required in each particular case, and it may be necessary to take measures to improve assay precision through modification of experimental procedures.
Discussion
Different approaches to assessing nonparallelism may be appropriate in the context of specific experimental situations. In many cases, the classic F-test can serve as a starting point for assessment of similarity, and it may be possible to evaluate the extent to which the underlying statistical assumptions for this test are satisfied. Application of an appropriate method and selection of appropriate criteria for assessment of parallelism depend on understanding the relevant properties of the biological test material, reference preparation, and assay system, as well as the purposes for which the result is required. Historical empirical data are often key to determining the approach, and wherever empirical approaches are considered suitable, they should be included in assay validation.
Sometimes practical and feasible assay designs may not meet the statistical assumptions required for the classic analysis of variance. In such situations, it may be possible to determine the impact of an assay design on the numerical statistical analysis. Underestimation of the residual error frequently results, with the consequence that an F value determined in the usual way is too large and hence appears to be significant. Assessment of deviations from parallelism (and hence the significance level) that may be expected on the basis of the assay design can be achieved by analyzing coded duplicate samples, as used for example in (13). In such situations, an equivalence-testing approach might also be appropriate. The EP indicates possible approaches to use when an assay design does not permit valid estimation of the relevant residual error from individual assays.
Table 1: Data for Figure 4, comparing F-test and equivalence testing, show levels set for confidence intervals of slope differences, results (intervals for slope differences), null hypotheses, and conclusions
Table 1: Da ta for Figure 4, comparing F-test and equivalence testing, show levels set for confidence intervals of slope differences, results (intervals for slope differences), null hypotheses, and conclusions ()
All these approaches are essentially empirical and depend on knowing the properties of the biological preparations and assay systems as well as supporting historical data. Although broad guidelines may be suggested, each situation and assay design is unique and must be individually evaluated. Moreover, all changes in experimental conditions or assay design would require revalidation.
Approaches to analyzing nonlinear dose–response relationships are more mathematically and statistically complex. The effects of the mathematical formulation selected — and of the constraints placed on the various parameters — must be evaluated (5, 14). The need is recognized for fundamental validity (for exact similarity of the dose–response relationships), although how to ensure that is less obvious. For example, the order in which multiple parameters are compared must be considered as well as the actions to be taken if one or more parameters differ significantly between the two curves.
Complete characterization of the dose–response relationship may be of primary importance when characterizing the biological material. However, for routine batch release of biologicals with well-characterized dose–response relationships, assay designs based on the linear part of the dose–response relationship may prove appropriate. This is particularly the case for animal-based assays, which commonly involve ethical and legal constraints on the number of animals used, thus limiting the number of data points that can be obtained. For any type of bioassay, cost or logistical considerations may limit the number of data points. In such cases, it frequently proves more useful to maximize the number of doses and the replicates of each dose over the linear part of the curve rather than attempting to span a complete dose–response curve. For the approximately linear part of the curve, a classic parallel line analysis can then be used.
The hypothesis tested by the classic analysis of variance for assays following the linear parallel-line model is based on the essential fundamental assumption of biological similarity. It is thus appropriate for assessing the validity of assays used to estimate the relative potency of identical preparations or those that behave identically in the particular assay system (and thus are functionally identical).
If the preparations are not functionally identical, then increased assay precision (subject to statistical validity of an estimate of precision) may indeed lead to increased rejection of assays. Improved resolution resulting from greater precision is always desirable. Improved precision will not resolve two materials that really are identical, but it will reveal previously unresolved differences, offering the possibility of exploring potential clinical significance of a newly detectable functional dissimilarity.
Failure to satisfy the classic “test for parallelism” (demonstration of significant deviations from parallelism) is thus a clear indication of an invalid estimate of relative potency. This invalidity may result from various causes, and it is important to recognize these causes and see that they are not obscured by the approaches used to assess nonparallelism. Such recognition can lead to alternative interpretations of the analysis or to alternative methods of analysis — and hence to estimates of potency that can be considered valid in a given situation.
It is not possible to “test for parallelism” because a hypothesis of exact equality can never be proven. Moreover, there is no single correct way to test for nonparallelism. As discussed by Fisher, no standardized method of analysis should be taken ready-made (10). It is important that details of each experimental situation are explicitly included in the analysis and interpretation of resulting data. In the case of bioassays, this includes considering the nature of the materials compared and the purpose of that comparison in addition to the assay design and statistical/mathematical characteristics of the biological response data. In no case can the requirement for fundamental validity of an assay and similarity of dose- response curves be ignored.
REFERENCES
1.) ICH Harmonised Tripartite Guideline Q6B.
2.) Lightbown, JW 1961. Biological Standardization and the Analyst: A Review. J. Soc. Anal. Chem. 86:216-230.
3.) Hauck, WW. 2005. Assessing Parallelism Prior to Determining Relative Potency. PDA J. Pharmaceut. Sci. Technol. 59:127-137.
4.) Callahan, JD, and NC Sajjadi. 2003. Testing the Null Hypothesis for a Specified Difference: The Right Way to Test for Parallelism. BioProcessing J..
5.) Plikaytis, BD. 1994. Determination of Parallelism and Nonparallelism in Bioassay Dilution Curves. J. Clin. Microbiol. 32:2441-2447.
6.) Song, Z. 2004. A Single Amino Acid Change (Asp 53→ Ala53) Converts Survivin from Anti-Apoptotic to Pro-Apoptotic. Mol. Biol. Cell. 15:1287-1296.
7.) Jerne, NK, and EC. Wood. 1949. The Validity and Meaning of the Results of Biological Assays. Biometrics 5:273-299.
8.) Lansky, D. 2002. Strip-Plot Designs, Mixed Models, and Comparisons Between Linear and Nonlinear Models for Microtitre Plate Bioassays in the Design and Analysis of Potency Assays. Dev Biol. 107:11-23.
9.) Cornfield, J. 1964. Comparative Bioassays and the Role of Parallelism. J. Pharmacol. Exper. Ther. 144:143-149.
10.) Fisher, RA. 1951.The Design of ExperimentsSixth Edition, Oliver & Boyd, London.
11.) Story, MJ. 1986. A New Parallelism Acceptance Criterion for Validating Large Plate Bioassay Results. J. Biol. Standardiz. 14:249-254.
12.) Klein, J. 1999. Validation of Assays for Use with Combination Vaccines. Biologicals 27:35-41.
13.) Robinson, CJ. 2006. The World Health Organization Reference Reagent for Keratinocyte Growth Factor, KGF. Growth Factors 24:279-284.
14.) Gottschalk, PG, and JR Dunn. 2005. Measuring Parallelism, Linearity, and Relative Potency in Bioassay and Immunoassay Data. J. Biopharmaceut. Stat. 15:437-463.