AI is stronger and faster. But is it better?
Industry will have to rethink GMP regulations to accommodate AI and ML, says a panel of experts.
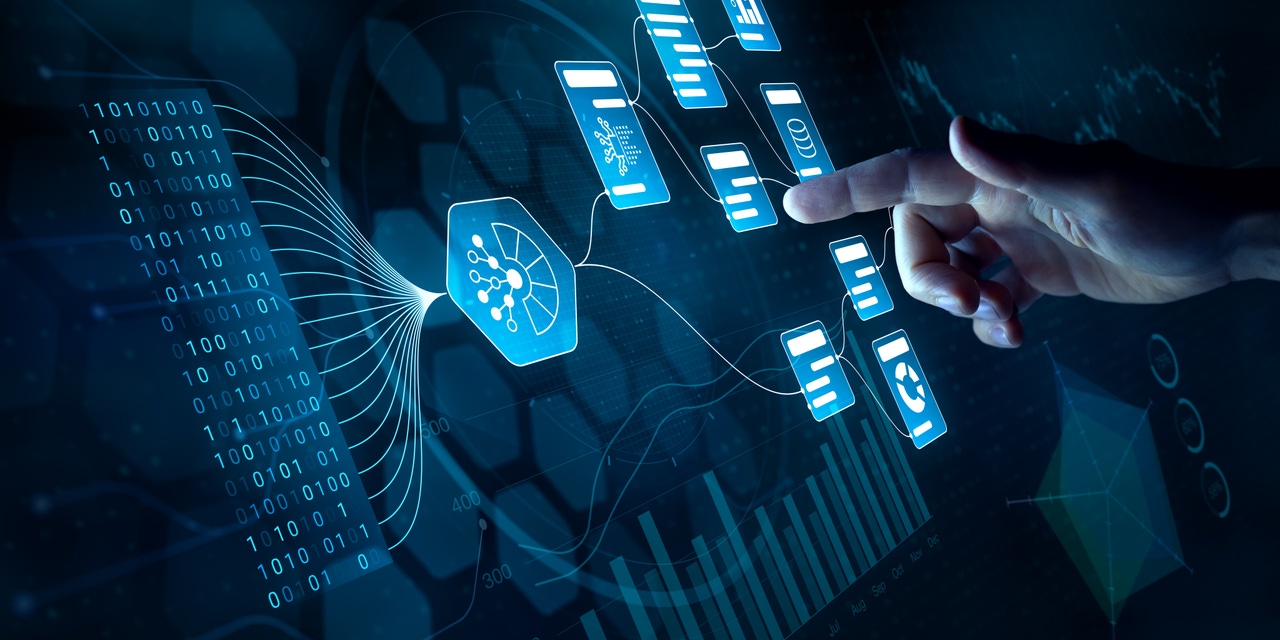
Fierce Pharma hosted a webinar earlier this month that delved into how quality systems can control artificial intelligence (AI) to enhance productivity. Bryan Ennis, president of AI-focused life sciences service firm Sware, moderated the discussion. He said that “as we think about how AI emerges within life sciences, it’s going to rub up against good manufacturing practice (GMP) regulations.”
AI is a broad term that encompasses multiple technologies including machine learning (ML), which Jamie Hijmans, chief technology officer (CTO) of regulatory-software developer Global Exponential Technologies, defined as a subset of AI. “There are two broad camps we can think about AI,” he said. One is augmentation-based AI that enables users to perform tasks faster and more accurately. The other is automation, which automatically generates an output based on a user input.
The emergence of AI in GxP manufacturing
AI technologies have found their footing in different parts of the bioprocess. “What we’re starting to see is a lot of use cases in the research and development space,” said Madhavi Ganesan, director, life sciences advisory at professional services network KPMG. But she added that quality control (QC) and manufacturing processes are sensitive from a good practice (GxP) perspective and are running behind in AI adoption.
“As we do move into the manufacturing space, I think there are a couple of aspects to consider,” added Michelle Vuolo, head of quality at manufacturing solutions firm Tulip Interfaces. She said that manufacturers need to consider the levels of autonomy that are present in different types of AI. She added it is important to have a human in the loop and to maintain risk-management approaches when using AI technology.
She also recommended that ML should be used with caution because of its ability to adapt without human intervention. “As you [implement] these self-changing algorithms, the risk becomes a little higher and might require more testing.” She said the International Society for Pharmaceutical Engineering (ISPE) has released guidance for risk-based approaches to using AI in GxP, with another document currently in the works.
Ensuring data quality
Ganesan emphasized the importance of using good data to train AI models. “If we don’t have good data governance, we can validate these systems until we turn blue, but the actual results will not be what we need.”
Hijmans agreed. “Models are created off of data, and the quality and representativeness of that data is deeply important.” He added that companies should investigate third-party software extensively before adopting it. “Only use systems [if you] understand how the system was trained and how data went into it. And if you can’t, investigate building your own.”
About the Author
You May Also Like