The product development team at a gene therapy contract development and manufacturing organization (CDMO) was working on a high-priority drug-substance project for a key client. The material was crucial to that client’s early stage clinical trial, with an immediate value over US$500,000 to both the client and the CDMO. Unfortunately, the bioreactor used in the upstream process — a transfection unit operation for an adenoassociated virus (AAV) vector — had developed an intermittent problem that could force it to shut down, killing the cultured cells.
To meet the clinical trial timeline, the production run had to move forward, and no other bioreactor was available. If the process scientist and technicians could catch the problem in time when it occurred, then they could diagnose it quickly and reset the unit with no ill effects on the cells. But the lead scientist could not be there 24 hours/day, seven days/week over the course of the multiple-day culture.
The CDMO’s automation-engineering team accelerated a technical solution to connect the unit with a remote monitoring and alarming system so that the lead scientist could be notified at home, evaluate the bioreactor status from a personal device, and provide instructions to after-hours technicians on site. During the run, the bioreactor did have multiple issues, but with the team able to address those problems in real time, the cell culture remained unaffected. At that point, the automation team had spent about $200,000 creating the infrastructure to enable the remote alarming and monitoring.
That success story made its way around the product-development and manufacturing-operations teams at the company. Soon, increased demand for implementing similar technologies elsewhere triggered discussions about what could be possible with remote access. The automation team quickly obtained approval to continue enhancing the initial solution they’d developed and to expand it to cover other process equipment. Over the following months, the remote capabilities implemented by that team saved multiple runs directly, mitigated deviations, and enhanced remote collaboration with clients and peers across the organization. The technology also enabled this CDMO’s overworked scientists, engineers, and operations leaders to breathe a little easier at home.
That story does not describe a reactive response to a problem. It was a planned “win” in a change-execution management (CEM) plan meant to enable incremental buildout of process intelligence capabilities for an organization over time while driving needed changes in behaviors to accomplish that goal.
Setting the Stage
Herein, I use process intelligence to describe the set of capabilities and supporting information needed to develop a new therapy, establish the necessary controls to monitor biomanufacturing, and ensure that such important information is made available in real time for product development, manufacturing, quality assurance, and other groups who need it.
Necessary capabilities include the following:
• remote process alarming and monitoring
• identification and monitoring of key aspects, including critical process parameters (CPPs) and critical quality attributes (CQAs)
• multiple crossbatch and crossproduct analytics
• continuous process verification
• bioprocess modeling
• enablement of straight-through processing.
The difficulty in making such capabilities a reality arises from the need to enable movement of process data around a company (Figure 1). Data are generated and owned by many stakeholders in research, quality, manufacturing, engineering, and even supply chain and finance teams. Each team will work to optimize its own processes and systems. Those teams need help to enable information flow to every stakeholder across the company.
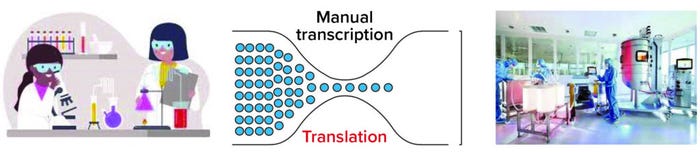
Figure 1: Multiple bottlenecks can slow the flow of information across teams. Automation and “digitization” help, but removing the need for data translation is key.
Getting started on the path toward these capabilities can be daunting — and changing people’s behavior can be the hardest part of the process. Below I outline how to get started and set a proper foundation for success without making multimillion-dollar bets up front on information technology (IT) platforms. The necessary capabilities can be established in simpler versions (a revision 1 instead of later versions) to deliver value earlier (Figure 2).
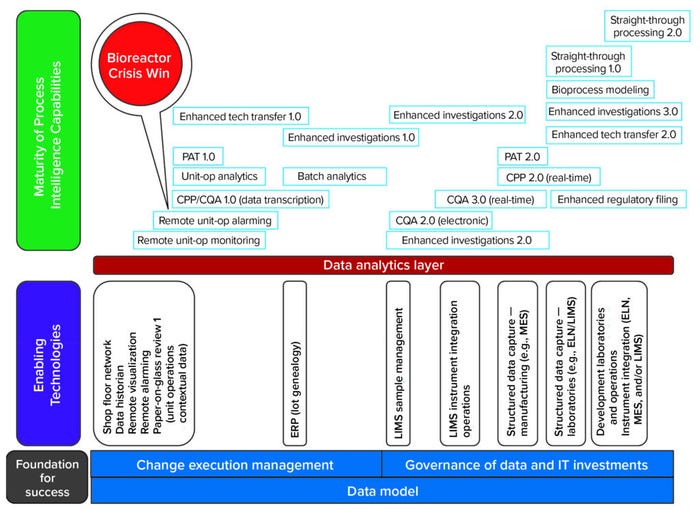
CPP = critical process parameters.
CQA = critical quality attributes.
ELN = electronic laboratory notebook.
ERP = enterprise resource planning.
LIMS = laboratory information management system.
MES = manufacturing execution system.
PAT = process analytical technology.
Setting a Foundation
In the CDMO case above, several key workstreams already were in play that allowed the teams to take advantage of the bioreactor crisis when it arose. Alignment on IT strategy existed with senior leadership, the necessary IT governance process was in place for making investment decisions, and key thought leaders already were in early discussions on the need for a data model and data governance.
Process Intelligence — An Integral Part of IT Strategy: The IT strategy was not about digitization (1); it was developed with the different business units and based on their prioritized capabilities. The applicable set of enabling technologies and steps in this case study is consistent for all large-molecule pharmaceutical companies:
• Start by building the necessary infrastructure to support laboratory and manufacturing systems.
• Initiate an enterprise resource planning (ERP) platform program.
• When resources are available, move on to other capabilities, such as laboratory information management systems (LIMS) and manufacturing execution systems (MES).
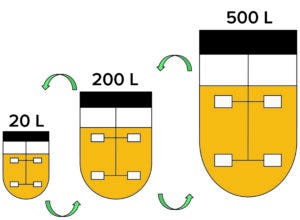
Figure 3: Information generated at each process scale needs to be shared for many reasons, and establishing consistent definitions across teams speeds that exchange. Such consistency had an immediate benefit for all parties in the case study by speeding the implementation of remote monitoring and alarming capabilities while setting a stage for future developments.
The CDMO’s IT and automation teams determined how they could build process intelligence capabilities in parallel with the implementation of enabling technologies (Figure 2). The company’s leadership team was open to that proactive approach based on experience. They had felt the pain of trying to pull data from disparate systems (e.g., ERPs and LIMS) that had been built independently. Management had witnessed critical scientific and engineering teams spend far too much of their time manipulating process data before they could even start applying that information to solve problems. So the leaders found value in establishing a foundation for sharing data at an early stage (Figure 3).
Each investment in an enabling technology would provide for data aggregation within the same architecture and across programs without translation. That could deliver capabilities providing immediate value to the business and provide “building blocks” for future developments that were laid out in an overall IT vision for the company (Figure 2).
The IT team already had put in the first elements of an isolated shop-floor network so that process and laboratory equipment could be accessed remotely with adequate security. The data historian and remote-access visualization and alarming tools were put in place and then connected to high-priority pieces of equipment.
When the team saved that first bioreactor run, however, the data historian and remote-access systems were not validated. The final design for the shop-floor network was not yet in place, and not all manufacturing equipment was connected to the network. But the team did not wait for a 100% solution to demonstrate the business value of a remote-monitoring strategy. That is to say, they did not let the perfect get in the way of the good. A “win” in the strategic plan did not need to be perfect; it needed to be functional at the time and to demonstrate the value of the strategy as a whole.
IT Governance in Place to Lead Decisions on Investments: The IT governance model (Figure 4) was established and agreed upon by representatives from all key areas. That was facilitated by IT and automation- engineering groups as part of establishing the company’s overall IT strategy, of which process intelligence was a key element.
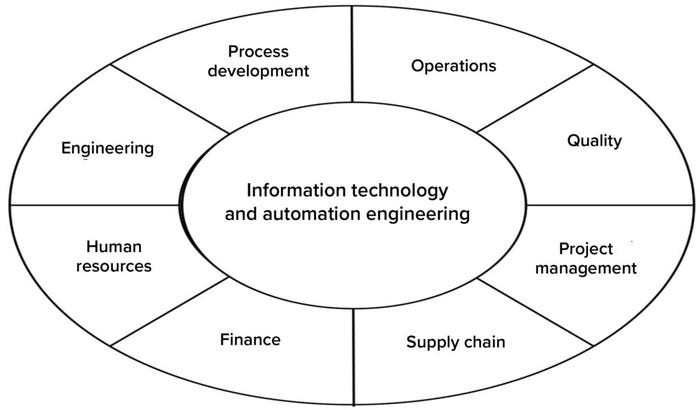
Figure 4: In this governance model for information technology (IT), representatives from all key functions help to frame the IT strategy and roadmap. Ongoing efforts include review and management of new demands according to the agreed-upon IT strategy, scoping of projects optimally across the organization, and prioritization of new investments.
In the CDMO case, this approach drove consistency in investments, enabling the business, IT, and automation teams to focus their resources appropriately (2). The team standardized to a single data historian and one type of remote access and alarming rather than using multiple systems or vendors siloed by department.
The team established an IT governance strategy early in the company’s development before disparate team cultures could “solidify” and complicate the drive for consistency. As departments get larger, their processes mature and become more internally consistent, which is a good thing in general. But the downside of that organic process is that if IT governance does not get set early on, then multiple systems could be performing the same function in different areas. The cost of shifting to common systems later on ultimately is more expensive in capital cost and especially in draining resources of subject-matter experts (SMEs). At an early stage in a company’s growth, the culture remains flexible enough to drive the necessary change with less effort, and the leadership team in this case study strongly supported a strategy of identifying common solutions.
Establishing the Data Model and Its Governance: The process engineering team already had discussed how to define a first-pass unit-operation configuration for the common production processes. But the bioreactor crisis highlighted a need to make consistent definitions for stakeholders across product development, manufacturing operations/engineering, and quality groups (Figure 3).
The data historian and remote access and alarming systems were configured in the same way for similar larger-scale unit operations in the CDMO’s biomanufacturing organization. Keeping the same format and presentation enabled teams to examine data easily across development at each scale — and facilitated the IT and automation teams’ ability to implement these capabilities quickly and easily.
After those events, a data governance team began to frame out the rest of the company’s data model in stages based on this experience. That first “win” helped them recognize the need for data consistency and what was possible regarding accelerating the flow of information for everyone.
Establishing a Change Execution Management (CEM) Plan: In an international best-selling book, Harvard Business School professor John Kotter and Deloitte Consulting principal Dan Cohen write, “People change what they do less because they are given analysis that shifts their thinking than because they are shown a truth that influences their feelings” (3). The process-intelligence strategy illustrated herein was received well right away by the operations and scientific leaders who needed to consume data. However, it did not offer obvious benefits to the people who generated the data. People need to know “what’s in it for me” (WIIFM) to make a change in their behavior. So the IT/automation team formed a CEM plan to generate “wins” and encourage commitment to the strategy among key stakeholders (especially data generators).
In this case study, talking about long-term data-analysis strategy would not have helped the team save the bioreactor run that was their immediate priority. After these events happened and remote monitoring and alarming use had become more commonplace at the CDMO, discussions on how the strategy could enable additional process-intelligence capabilities began to make headway with people who generate data.
Stories about runs saved, operational problems solved, and the need for investigations mitigated went around the company by word of mouth as well as through the CEM communications-plan deliverables. Product development and manufacturing operations teams then could see value in the implemented strategy to themselves and the company overall. Key scientists and engineers could get home on time for dinner, eliminate a great deal of paperwork, and focus more of their time on advancing new therapies. Conversations on process intelligence and data governance changed after this experience. The questions were no longer why/what but rather how/when. A number of SMEs asked how they could help.
A Foundation for Success: After the success described above, the CDMO’s IT and automation teams were aligned strongly with the business units they served and had a clear process for making and prioritizing technology-investment decisions. The “win” had generated credibility for IT’s strategic plan and provided solid financial data to support continued investment in the future.
Consistent unit-operation definitions in the data model set a foundation for the company to build out “paper on glass” batch-data collection of CPPs in real time and tie those results together with data coming from the automated systems. That could provide a remote, real-time picture of run status for a unit operation in progress. When IT initiated the ERP project, alignment to the process intelligence strategy and the data model were core requirements applied to it.
That strategy would enable readily connecting the raw materials of each unit operation before putting a costly MES into place. Figure 2 illustrates the strategy of enabling a team to achieve version 1.0 of enhanced investigations by standardizing data definitions across systems — before the more capable version 3.0 of enhanced investigations aided by the additional MES capability. Such data standardization also increases the speed of MES deployment while reducing costs.
One Step at a Time
Lao Tzu said, “The journey of a thousand miles begins with a single step.” The example herein of a company getting started on its journey toward process-intelligence capability shows how that applies to biomanufacturing. The CDMO began by focusing on protecting high-value bioreactor runs. But the company could have started with a different priority, such as speeding throughput for analytical development and/or the quality control laboratories. The same approach can be applied to any starting point, but the foundational elements needed for process-intelligence success remain the same for organizations involved in biopharmaceutical research and/or manufacturing.
References
1 Lewis B, Kaiser D. There’s No Such Thing As an IT Project: A Handbook for Intentional Business Change. Berrett-Koehler Publishers: Oakland, CA, 24 September 2019.
2 Weill P, Ross JW. IT Governance: How Top Performers Manage IT Decision Rights for Superior Results (1st Edition). Harvard Business Review Press: Brighton, MA, 1 June 2004.
3 Kotter JP, Cohen DS. The Heart of Change. Harvard Business Review Press: Boston, MA, 2002.
Dermot McCaul is owner and principal consultant at McCaul Consulting LLC in Philadelphia, PA; [email protected].