NIR Spectroscopy for Process Monitoring and Control in Mammalian Cell Cultivation
The quality by design (QbD) and process analytical technology (PAT) approaches have shown significant benefit in the classical pharmaceutical industry and are now strongly influencing bioprocessing. Monitoring critical process parameters (CPPs) during biotechnological cell cultivations is essential to maintaining high efficiencies and quality. Commercial sensor systems for real-time inline monitoring are available for some parameters, such as pH or the concentration of dissolved oxygen (DO). For others such as glucose concentration, total cell count (TCC), and viability no robust online prediction is yet possible for most applications. This gap may be closed with the help of near-infrared spectroscopy (NIRS), which can provide quantitative prediction of single analytes with real-time measurement.
PRODUCT FOCUS: RECOMBINANTproteins
PROCESS FOCUS: Production
WHO SHOULD READ: QA/QC, process development, and manufacturing
KEYWORDS: Quality by design, process analytical technology, statistical analysis, robustness, reproducibility
LEVEL: INTERMEDIATE
Photo 1:
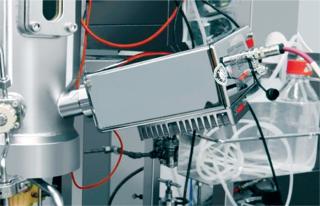
Photo 1: ()
For accurate process control based on NIRS, special care has to be taken while building a calibration model (1, 2). In cell cultivation, most analytes are confounded and show large correlation coefficients. Therefore, partial least squares (PLS) regression models cannot discriminate between the signals of different analytes. Analytes confounded with major changes in NIR spectra (usually caused by cell growth) need especially careful evaluation. Spiking experiments are the most efficient ways to break correlations between critical analytes such as glucose and other nutrients.
That strategy should be followed to build robust calibration models without correlations (3, 4). Another very critical issue occurring in cell cultivation are batch-to-batch variations. Full cross-validation models cannot be applied for predictions in cell cultivation because most batches are part of both the calibration and validation set. Accuracy of new batch predictions cannot be estimated using that approach (5). The evaluation procedure recommended in good modeling practice (6) is to use several complete and “unseen” batches for model validation.
Use of multivariate statistical process control (MSPC) models in conjunction with NIRS is gaining importance in process control (7, 8). This approach does not rely on reference methods or predict analyte concentrations. Rather, it focuses on describing the whole process and deviations to desired behavior. In particular, batch trajectories (or batch evolution models, BEMs) represent an easily comprehended, online, good-or-bad assessment of a batch in progress. It allows operators to identify deviations and counteract them immediately.
In addition to offering quantitative analysis, MSPC is a particular strength of NIR spectroscopy. In terms of MSPC, spectral overlap of information in combination with strong confounding of analyte profiles constitute an advantage that provides optimal prerequisites for low noise and robust models. For MSPC modeling, process variations can be introduced for evaluating to which extent the spectra reflect changes within a process operation.
Here we describe our evaluation of a new free-beam NIR spectrometer (the BioPAT Spectro instrument from Sartorius Stedim Biotech) for monitoring and control of Chinese hamster ovary (CHO) cell cultivation processes. To address calibration issues such as confounding and batch-to-batch variances, we collected and analyzed more than 300 samples for eight runs in total. Additionally, we conducted spiking experiments with glucose and glutamine to separate the results from cell count. And finally, we introduced process variations in glucose limitation and changes in the aeration strategy. This provided an excellent data set for evaluating the possibilities and limits in quantitative predictions of analytes during cell cultivation using NIRS. With it, we could generate robust models involving up to six calibration batches that can be tested against at least two complete batches in a validation set. In terms of MSPC, BEMs can be evaluated for their sensitivity to process deviations.
Material and Methods
We cultivated CHO-K01 cells (cell culture technology department, University of Bielefeld, Germany) in a BIOSTAT C plus bioreactor (Sartorius Stedim Biotech, Germany) at the Institute of Technical Chemistry (Leibniz University of Hanover, Germany) with a 7.5 L working volume. The initial seeding density was 4 × 105 cells/mL, and the culture medium was TC-42 from TeutoCell AG). We added glutamine (1 g/L final concentration) and antifoam (Pluronic F68, 4 mL of 10% solution per liter of medium) before inoculating the cells. The process set points were as follows: 37 °C, pH 7.1, agitation rate 200 rpm. We set the maximum aeration rate to 0.5 L/min (air) or 0.3 L/min (air–oxygen mixture) to regulate dissolved oxygen at 40%. In total, we performed eight cultivation runs, each lasting an average of six days.
During and after the batch phase, we fed glucose or spiked analytes in five of these batches to introduce process variability or to break correlations between analyte concentration profiles. Three fed batches were performed with optimal process parameter settings to evaluate process repeatability. We used spectra from those batches for golden batch modeling in MSPC. And we introduced process variances by limiting the maximum aeration rate to 0.5 L/min of adapted air–oxygen mixture, causing a higher cell growth rate. We sampled the cultures every three to six hours and determined using laboratory reference analytics of CPPs such as total cell count (TCC), viability (measured with a TC10 automated cell counter from Bio-Rad Laboratories), glucose, lactate, glutamine, and so on (using a YSI 2700 bioanalyzer from YSI Inc.).
NIR spectra were measured online throughout the entire cultivation time using SX-Suite software and a BioPAT Spectro free-beam NIR spectrometer from Sartorius as a process analyzer in the range of 1,050–1,650 nm. To withstand clean-in-place and sterilize-in-place (CIP, SIP) cycles, this process analyzer is adapted with a 25-mm standard Ingold port. The fiber-free port adapter holds a flow-through transflection cell with a large spot size (21 mm) that enables robust and low-noise signals. Spectra for multivariate calibration averaged over 60 seconds. We ran multivariate data analysis of the NIR spectra using the SX-System Plus program to predict critical process parameters.
Using SIMCA 13 software from Umetics, we carried out our MSPC data evaluation. BEMs display major variances within a process over time based solely on spectral data, and they were produced using a subset of batches showing optimal performance. The process yields a golden batch trajectory as well as process limits calculated from the standard deviation obtained from each time point. For model
validation, we compared unseen batches with our model. Statistically relevant deviations from optimal behavior appear when a validation batch exceeds the limits of a process.
Results
For quantitative analysis, we used a PLS algorithm to predict CPP concentrations correlated with laboratory reference measurements. To obtain predictive estimates reflecting the situation of a new batch as closely as possible, we do not use internal validation (cross-validation or internal test set calibration). Instead, all calibrations involve a subset of complete batches that are not part of the validation set, and validations involve batches that aren’t in the calibration set.
Table 1 overviews the models and accuracy of predictions for several analytes investigated. We obtained an excellent model for TCC using two principal components. No data pretreatment was needed. The standard error of prediction (SEP) is 0.48 in a range of 0–7 × 106 cells/mL. Figure 1 (TOP RIGHT) shows the estimate plot for the combined calibration and validation set of TCC. Deviations increase with TCC, which can be ascribed to higher uncertainties in the reference method for the higher cell counts. The accuracy of cell count prediction based on NIR spectroscopy is obviously limited by the accuracy of the reference method. Figure 1 (TOP LEFT) shows the prediction of a complete batch that was not part of the calibration set. That external validation result can be seen as a prediction because it can be expected from any completely new batch. The predictions (green line) perfectly match the reference values (green circles).
Table 1:

Table 1: 194; ()
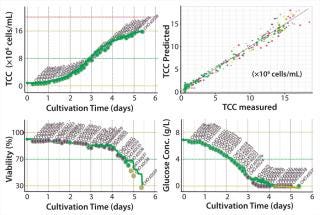
Figure 1: ()
Viability can be predicted with an SEP of 4.2 in a range of 28–100%. Four factors are needed for the viability model. Predictions of viability within an unseen batch follow the reference values’ profiles exactly up to 70%. Deviations are seen for lower viability values because only reference values are available in the low range.
Glucose can be predicted in a range of 0–8 g/L with an SEP of 0.48 using four principal components. We reduced correlations of glucose with other analytes by spiking it in one cultivation, to which glucose was added in three steps up to 9 g/L after the batch phase. In three other cultivations, we fed glucose into the process after the batch phase, keeping the glucose level at 1–3 g/L for the rest of the process time. Both approaches significantly decreased the correlation coefficient with other parameters such as TCC.
Low-concentration analytes such as glutamine also seem to be predictable at the first glance, but they strongly correlate with other parameters (e.g., TCC). We don’t recommend using models based on correlations for process control because they show a lack of both robustness and sensitivity to the analytes of interest. Whether a model is based on correlations can be easily determined with spiking experiments. For example, we spiked glutamine in one cultivation during the exponential growth phase up to 1 g/L. The glutamine model could not predict the spiking, which proves its strong correlation with other analytes. So glutamine cannot be measured directly in this concentration range using NIRS. Qualitative models on overall nutrient consumption or metabolite accumulation do yield promising results, however (data not shown).
Additional benefit comes from MSPC of NIR data. In our work, we generated batch trajectories from major variances of the NIR spectra. Therefore, the score values were used and plotted over time using SIMCA 13 software. Figure 2 (TOP) shows our BEM build for the first principal component of the NIR spectra. Three batches contribute to this model, which shows optimal cell growth. All batches show a nearly identical profile, indicating high batch-to-batch reproducibility both in terms of process operation and spectra acquisition. We call the mean trajectory (green dashed line) a “golden batch,” which represents the profile of optimal performance. Process limits (red dashed lines) can be calculated by three times the standard deviation of the batches involved in the model. Other batches can be compared with the model. As long as the trajectory of a new batch stays within limits, it can be designated as statistically identical to the golden batch. A relevant process deviation is flagged if the trajectory strays outside those limits.
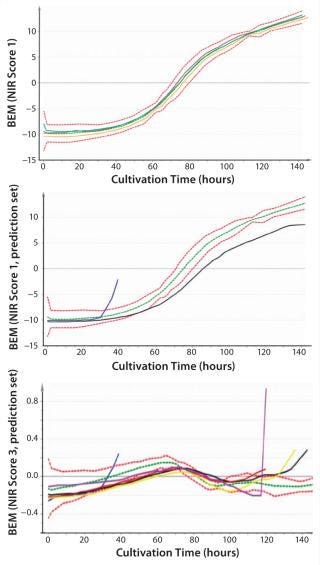
Figure 2: ()
Figure 2, MIDDLE shows significant process deviations. The trajectory of batch 3 (blue line) surpasses process limits after 30 hours because of a bacterial contamination during the process. In batch 2 (black line), a different aeration strategy resulted in a lower cell growth rate. That can be easily identified by the low performance of the first principal component trajectory. The trajectory built from the first principle component of the NIR spectra clearly provides a qualitative estimation about TCC.
Figure 2, BOTTOM shows a BEM based on the third principal component. This model (dashed lines) is again generated from high-performance batches, as seen in the model above. As already seen in the model from the first principal component, contaminations also can be identified (violet and blue batches) in this model. Two other batches (black and yellow lines) exceeded the process limits after 130 hours. In both batches, viability dropped earlier than in the high-performance batches. After the initial batch phase, no sugar was added in batch 2 and 5 as it was done in the high-performance batches. That early glucose limitation caused an early drop in viability, which was also proven by reference analysis. The large deviation at the end of batch 7 is also correlated with a sudden drop of viability (from 90% to 10% verified by reference analysis).
Trajectories based on the first and third principal compo
nents both show important trends within a cell cultivation process linked to cell parameters such as TCC and viability. Those trends can be followed without conducting laborious reference analytics.
Our Conclusions
The Ingold-port adaption of a free-beam NIR spectrometer is tailored for optimal bioprocess monitoring and control. The device offers an excellent signal-to-noise ratio, a large free aperture, and therefore allows for large sample volumes. That can be seen particularly with our batch trajectories showing high reproducibility.
Quantitative analysis is possible in a multiparameter approach for TCC, viability, and glucose in real time. But the accuracy of TCC prediction (SEP = 0.48 × 106 cells/mL) is limited by the error of the reference method. Major variances within the NIR spectra derive from scattering effects at cell surfaces and are therefore linked to TCC. That may also include variances due to changes in morphologic properties of cells during apoptosis, which could be the reason for the strong correlation of the third principle component with viability. Clearly, cell parameters can be monitored with high accuracy with free beam NIR spectrometer.
Glucose concentration showed changes in the range of 0–8 g/L and could be predicted with an accuracy of 0.48 g/L. Spiking glucose caused significant changes within the spectra, which proves NIRS sensitivity for glucose in that concentration range. At much lower concentration ranges, other analytes (e.g., glutamine) cannot be predicted solely based on an NIR signal derived from the analyte itself. That was verified by spiking experiments.
In addition to single analytes, users can obtain promising predictions for sum signals such as overall metabolite accumulation. This provides useful information about the metabolic stage of a process, leading to a more accurate estimation of stage transitions. For all analytes, we validated the model by using batches that were not part of the calibration dataset. That was crucial for guaranteeing model robustness and revealing the limits of NIR spectroscopy in cell cultivation. As proven by spiking experiments and the introduction of process variability, cross-validation techniques are unsuitable for high-correlation batch processes such as this.
In addition to quantitative predictions, NIRS provides additional benefit through MSPC. Process trajectories based on major spectral variances show in easily comprehended graphs an online good–bad estimation of a current batch. Statistically relevant deviations from optimal performance can be detected immediately, providing operators with early fault detection. On one hand, this provides a far better process understanding and enables process evolution with small batch-to-batch variations. On the other hand, adjusting process parameters is made possible by real-time overview of processes, which can help to keep them within required specifications. That could lower the number of failed batches with immediate adjustment of CPPs.
Figure 3 overviews the benefits of using free-beam NIR spectroscopy in cell cultivation. Equivalent results — both for quantitative analysis and MSPC — could be obtained for cell lines producing monoclonal antibodies as well as bacterial fermentation (data not shown).
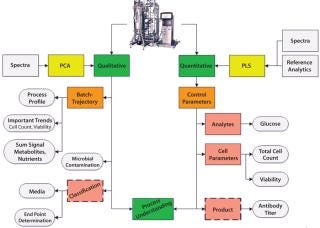
Figure 3: ()
Improved Process Understanding
Robust free-beam NIR process analyzers are indispensable tools within the PAT/QbD framework for real-time process monitoring and control. These instruments enable multiparametric, noninvasive measurements of analyte concentrations and process trajectories. Free-beam NIR spectrometers are ideal tools for defining golden batches and process borders in support of QbD. Moreover, sophisticated data analysis — both quantitative and MSPC — provides far better process understanding. Information can be accessed online in easily interpreted graphs that help operators make fast and knowledge-based decisions. All of this ultimately leads to higher stability in process operation, better performance, and fewer failed batches.
About the Author
Author Details
Marko Sandor, Ferdinand Rüdinger, Dörte Solle, and Thomas Scheper are with the Institut für Technische Chemie at Leibniz Universität Hannover, Callinstraße 5, D-30167 Hannover, Germany. Roland Bienert and Christian Grimm are with Sartorius Stedim Biotech GmbH, August-Spindler-Straße 11, D-37079 Göttingen, Germany; 49-551-308-3475, fax 49-551-308-2062; [email protected]. BioPAT Spectro and BIOSTAT C plus are registered trademarks of Sartorius Stedim Biotech, and Ingold is a registered trademark of EMD/Merck Millipore.
REFERENCES
1.) Cervera, A, and N. Petersen. 2009. Application of Near-Infrared Spectroscopy for Monitoring and Control of Cell Culture and Fermentation. Biotechnol. Progr. 25:1561-1581.
2.) Rodrigues, LO. 2008. The Use of NIR As a Multi-Parametric In Situ Monitoring Technique in Filamentous Fermentation Systems. Talanta 75:1356-1361.
3.) Arnold, SA. 2003. In-Situ Near Infrared Spectroscopy to Monitor Key Analytes in Mammalian Cell Cultivation. Biotechnol. Bioeng. 84:13-19.
4.) Vaidyanathan, S. 2001. Assessment of the Structure and Predictive Ability of Models Developed for Monitoring Key Analytes in a Submerged Fungal Bioprocess Using Near-Infrared Spectroscopy. Appl. Spectr. 55:444-453.
5.) Hakemeyer, C. 2012. At-Line NIR Spectroscopy As Effective PAT Monitoring Technique in MAb Cultivations During Process Development and Manufacturing. Talanta 90:12-21.
6.) Henriques, JG Rao, G. 2010.Monitoring Mammalian Cell Cultivations for Monoclonal Antibody Production Using Near-Infrared SpectroscopyOptical Sensor Systems in Biotechnology, Springer Berlin Heidelberg:29-72.
7.) Machin, M, L Liesum, and A Peinado. 2011. Implementation of Modeling Approaches in the QbD Framework: Examples from the Novartis Experience. Europ. Pharmaceut. Rev. 16:39-42.
8.) Undey, C. 2012. PAT In-Depth Focus. Europ. Pharmaceut. Rev. 17:22-29.
You May Also Like