A Methodology for Identifying Current and Future Skills Gaps: Future-Proofing the Bioprocessing Sector As It Embraces Industry 4.0 Principles, Part 2A Methodology for Identifying Current and Future Skills Gaps: Future-Proofing the Bioprocessing Sector As It Embraces Industry 4.0 Principles, Part 2
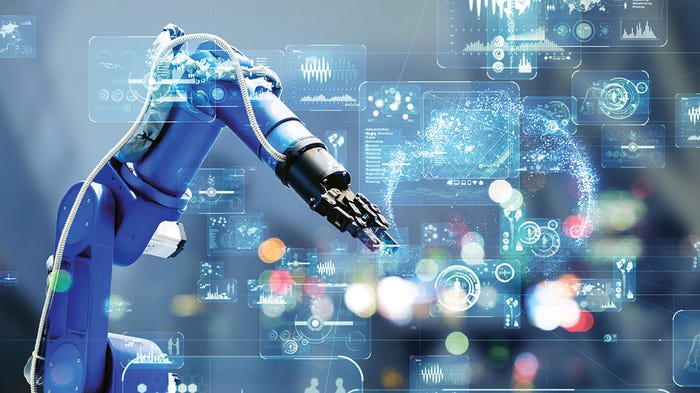
HTTPS://WWW.ISTOCKPHOTO.COM
The aims of our research have been to define and identify the specific expertise and skills gaps relevant to the biopharma 4.0 transition and to help the industry implement strategies to mitigate operational disruptions in the manufacturing of critical drug products. To do so, we used the Lightcast labor analytics database to identify real-time labor-market trends and the occurrence rate for specific skill and expertise terminology, executing a customized search by applying different filters.
Part 1 of this report described the biopharmaceutical industry’s transformative shift with the adoption of industry 4.0 principles. We identified existing key skills in the sector and the emergence of alternative skills that will be required to harness automation and digitalization. And we characterized the hypothesized skills gap as a critical challenge for which the complexities of biopharma 4.0 must be navigated to provide solutions. A “Methodology” section illustrated how we determined the skills gaps, primarily applying programming tools to the Lightcast and LinkedIn databases, categorizing data by job-posting keywords. We categorized, classified, and organized profile and job-posting data collected over five years and assessed skills development over 20 years. Data collection is ongoing to assemble up-to-date expertise, skills, and domain knowledge requirements from the Lightcast Jobs Posting Analytics (JPA) module and professional profiles data from the Lightcast Profile Data (PD) module. In addition, we’ve supplemented those data with information from LinkedIn and university databases.
Beginning in Part 1 and concluding here in Part 2, our “Results” section first provides a summary of the skills/expertise gap at macro (technology clusters) and micro (specific expertise) levels, outlining the evolution of supply and demand relevant to skills needed in the biopharmaceutical industry. The relationship between talent and job frequency in different technology clusters highlights the availability and demand for specific skills. Part 2 also includes discussion and conclusion sections to elucidate current, emerging, and future trends within biomanufacturing skills and expertise, with a summary of our findings and the limitations of this research.
Results, Continued
Talent Demand: By cross-referencing information from the Lightcast database with data from LinkedIn, we compared biomanufacturing talent frequency across the biopharma 4.0 technology clusters of automation, digitization, data science, cyber, and sensor areas. We chose those segments to cover generally the key talent involved in the biomanufacturing sector, supported with evidence of biopharma 4.0 adoption. We defined talent frequency as the available talent pool or 2023 talent supply for comparison against job postings using similar search criteria to delineate talent demand. We identified subdivisions based on our own expertise, so they do not reflect a collective categorization. Our aims were to represent biopharma 4.0 themes at a macro level and to identify and collate the talent supply among biomanufacturing companies, illustrating evidence of technology adoption as defined by the boundaries of biopharma 4.0.
Figure 6 illustrates that the current biomanufacturing talent exhibits strong expertise in automation (specifically for continuous biomanufacturing, CB). Data-science results show evidence of Python programming skills in addition to cybersecurity and optical/spectroscopy sensors. Note the lack of experience detected in virtual reality, artificial intelligence (AI), and digital twins. The figure also illustrates the frequency of unique job postings in US biomanufacturing segmented by biopharma 4.0 technology clusters. Automation appears with significantly higher frequency (69%) than do other technology clusters, suggesting that currently available roles require automation experience, with data science at 27% of biopharma 4.0 roles. Few job postings included an expertise requirement for cyber, sensor, or digitization capabilities.
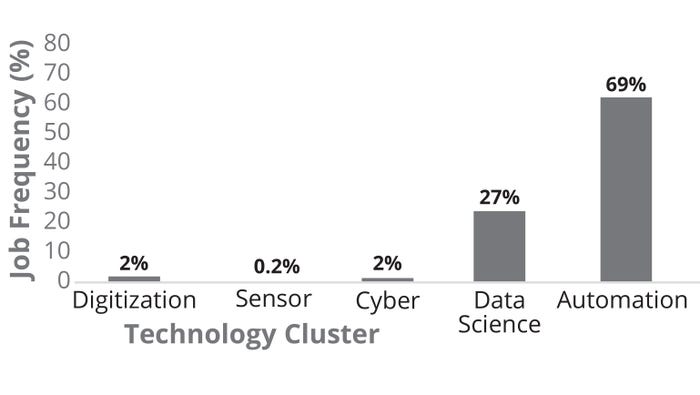
Figure 6: USA biomanufacturing job frequency (%) by biopharmaceutical technology clusters (2023).
For each segment, we identified specific keywords related to skills and expertise from the Lightcast database cross-referenced with data from LinkedIn. Table 1 in Part 1 outlines examples of biopharma 4.0 skills and expertise acquired from keyword searches for unique job postings. Here in Part 2, Figure 7 summarizes expertise frequency and therefore talent supply at a micro level.
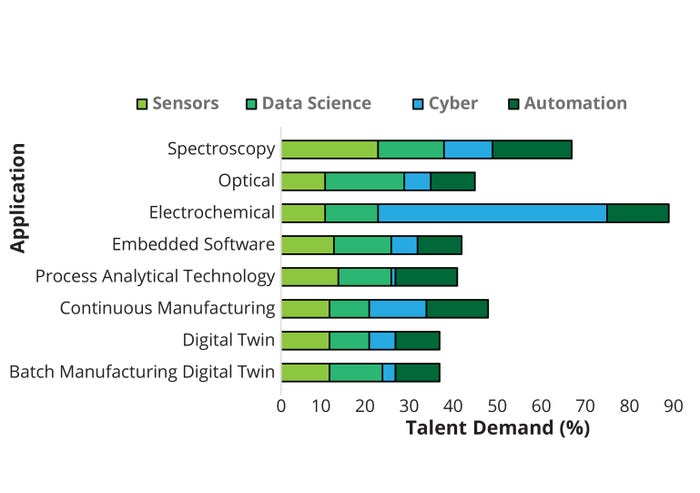
Figure 7: Talent demand by employee task application (micro level).
Automation: Keywords in this technology segment include automation, robotics, virtual reality, processing technology, lean manufacturing, simulation, root-cause analysis, quality management, and CB (Figure 7). Most job postings citing automation experience specifically relate to CB. Many cited quality, lean manufacturing, and root-cause analysis. Some roles also required virtual reality (VR) experience (25).
No specific expertise or skill set appeared frequently, suggesting that many distinctive role functions are incorporated into designated, specialized jobs. Automation and quality jobs particularly play critical biomanufacturing roles to ensure efficient and high-quality production processes. A common job role in these areas is “automation engineer/scientist,” someone who is responsible for designing, implementing, and maintaining automated systems and processes in biomanufacturing as well as developing strategies for process automation and robotics integration. Such professionals typically have expertise in industrial automation technologies and programming methodologies such as supervisory control and data acquisition (SCADA) and programmable logic controllers (PLCs), in addition to a deep understanding of bioprocessing operations. Other roles include process-control engineers, who focus on optimizing and maintaining control systems in biomanufacturing facilities; quality assurance (QA) specialists, who ensure that biomanufactured products meet regulatory requirements and quality standards; and validation engineers, who are responsible for ensuring that manufacturing processes and equipment meet predefined quality standards and regulatory requirements (48).
Those are just a few examples of the job roles related to automation and quality in biomanufacturing. As the industry continues to evolve, other roles and specializations may emerge within this area. Individuals with the relevant skills, knowledge, and experience of automation, quality control (QC), process optimization, and regulatory compliance will be in great demand to ensure efficient and quality-driven biomanufacturing operations.
Data Science: This technology segment includes skills and experience containing the keywords data science, data mining, big data, AI, machine learning (ML), and mathematical and statistical skills. Many job postings citing data-science experience specifically relate to programming skills, particularly R (18%) and Python (15%). A similar expertise frequency was detected for AI, statistics, and ML, all of which were popular requirements in biopharma 4.0 job postings (Figure 7).
As technology plays an increasingly prominent role in optimizing processes and analyzing data, computer programming skills are becoming more valuable in biomanufacturing than ever before (45). Conventional biomanufacturing job roles for which computer programming skills are beneficial include that of bioprocess engineer. Such experts develop software tools and algorithms to model, simulate, and optimize bioprocesses using programming languages such as Python, R, or Matlab to create mathematical models. Programming skills enable those experts to automate data analysis, visualize results, and implement advanced process monitoring and control systems.
Bioinformatics specialists apply computer programming skills to interpret biological (e.g., genomic, transcriptomic, and proteomic) data generated in biomanufacturing processes. Programming skills are essential for data mining, pattern recognition, and data visualization, which enable bioinformaticians to derive insights from large data sets and contribute to process optimization and product development.
Automation engineers use programming skills to design, develop, and implement automation systems in biomanufacturing. These experts program and integrate robotic systems, control algorithms, and data-acquisition systems — using languages such as Python, Java, or C++ — to optimize workflows and develop software solutions for process control and monitoring. In addition, software engineers, instrumentation and control engineers, and computational biologists apply similar programming skills to develop and implement computational models and algorithms for studying biological systems.
Those are just a few examples of how computer-programming skills are valuable in different biomanufacturing job roles (49). The absence of such specific expertise and skill sets noted frequently overall implies that many distinctive functions are incorporated into designated, specialized jobs. The job postings from biopharma-4.0–ready companies are for data scientists, who collectively play a vital role in biomanufacturing by applying programming skills to analyze and interpret bioprocess data. Such experts are very much in demand (Figure 7).
Cyber: Keywords including security, cloud computing, agile, Internet of Things (IoT), virtual, web, solution architecture, and development opportunities define the cyber segment. Skill sets predominantly revolve around the IoT, cloud computing, and cybersecurity. Most cyber expertise detected in biopharmaceutical job postings was specific to the keyword agile, with a frequency of 52% noted (Figure 7). “Agile” methodologies can be applied in biomanufacturing processes to enable rapid adaptability and responsiveness. By breaking down complex projects into smaller, more manageable tasks, teams can work in short iterations to deliver incremental improvements and respond to changing requirements. Agile biomanufacturing facilitates continuous process improvement, faster decision-making, and the ability to respond to market demands (49).
Agile and cybersecurity intersect with biomanufacturing. Agile methodologies such as “scrum” or “kanban” promote flexible approaches to project management and product development. Agile practices can be used to manage product-development life cycles, optimize manufacturing processes, and improve project execution. As the sector becomes increasingly digitalized, cybersecurity becomes of paramount importance. Protecting sensitive data, intellectual property, and critical infrastructure from cyberthreats is essential. Robust cybersecurity practices such as access controls, encryption, network monitoring, and incident-response protocols are implemented commonly to safeguard biomanufacturing processes. Secure software development incorporates agile methodologies throughout the development life cycle. By integrating security practices into agile workflows, companies can ensure that software applications, automation systems, and digital solutions are built with security in mind. In addition, agile methodologies and cybersecurity practices are both critical to proactive risk management as well as to compliance and regulatory adoption (50).
Consider that the frequency of cybersecurity, IoT, and VR skills in the job-postings data is low compared with the high frequency of “agile,” suggesting that many disparate roles were detected. In biomanufacturing’s evolution to industry 4.0, several job roles require a combination of agile methodologies and cybersecurity expertise. Examples include agile project managers, scrum masters, agile biomanufacturing engineers, cybersecurity analysts, agile data analysts, agile QA engineers, and software development and information technology operations (DevOps)engineers. Those are just a few examples of job roles in biomanufacturing that require proficiency in both agile methodologies and cybersecurity. As processes become increasingly digitalized and interconnected, the integration of agile with cybersecurity becomes vital to driving innovation, optimizing processes, and protecting critical assets in a rapidly evolving biopharma 4.0 landscape (23).
Figure 7 illustrates our assessment of the current biomanufacturing job postings, showing a strong requirement for automation experience — specifically related to CB, quality, lean manufacturing, and root-cause analysis. In addition, data-science experience (specifically programming skills in R and Python) is in high demand. Most cyber expertise detected in biopharmaceutical job postings was agile-related, and job postings for sensors were specific to spectroscopy.
Sensor: Keywords in this technology segment include process analytical technology (PAT), embedded software, digital twin, spectroscopy, electrochemical, optical, and continuous and batch manufacturing. Such skills are related mostly to sensor analytics and signal processing. A high percentage of expertise was detected in job postings specific to spectroscopy sensors (22%). All other skills were measured at ~10–13%, with no specific expertise or skill set appearing at high frequency — again suggesting that many distinctive functions are incorporated into designated, specialized jobs (Figure 7).
Biopharma 4.0 roles that require expertise in sensors and spectroscopy include sensor engineers/scientists, who develop and implement sensor technologies for real-time process monitoring, particularly in areas related to temperature, pH, dissolved oxygen (DO), pressure, and flow rate. Such roles involves selecting appropriate sensor types, integrating them into bioreactors or other process equipment, and developing calibration and validation procedures.
Sensor engineers/scientists work closely with process engineers to ensure accurate and reliable data extraction for process optimization. Spectroscopy analysts use techniques such as UV-vis, infrared, Raman, and fluorescence spectroscopies to analyze the composition, structure, and properties of biologically manufactured products and intermediates. Process-development engineers leverage sensor technologies and spectroscopy to optimize and scale up bioprocesses. Additional biomanufacturing roles with overlapping skills and expertise include automation engineers/scientists, QC analysts, and research scientists/engineers, all of whom use sensors and spectroscopy for process optimization, product characterization, and bioprocess monitoring (51).
Skills Gap: To assess potential biopharma 4.0 skills deficits, we analyzed job postings and talent profiles to conduct a gap analysis between the expertise available (supply) and the skills required (demand). Supply and demand data from 2018 to 2023 were collected (see Part 1) at both national (United States) and international (Europe, South Korea, and China) levels. For each profile and job posting, we obtained the skill requirements and recorded the most frequently mentioned skills. In addition, we analyzed the overall number of job postings and relevant profiles. Our gap analysis compared the occurrence rate of each skill in the supply and demand data to identify and measure the biopharma 4.0 skills gap.
We detected an abundance of mature and experienced talent matching the skills demand for automation, cybersecurity, and sensors. Specific expertise was absent for agile methods, VR, AI, and digital twins. However, sufficient automation expertise was present in the talent pool, where the required expertise for biopharma 4.0 can be achieved through development and learning.
The relationship between talent and job frequency in different technology clusters highlights the relative availability and demand for specific skills (Figure 8). Digitization and sensor areas have low talent frequency and limited job opportunities, indicating potential talent shortages and corresponding underuse of the technologies. The cyber area demonstrates a balanced alignment between talent and job frequency. Data science exhibits a higher talent frequency than do the digitization and sensors clusters but falls short of meeting the strong demand for professionals in the field, indicating a potential talent gap. Automation shows a high talent frequency and strong job demand, suggesting a well-matched supply and demand relationship. From our pilot-study results, a potential skills gap seems to exist for data-science expertise requirements, with 27% of job postings requiring data-science skills and only 14% of the talent pool found to be synergistic with that demand.
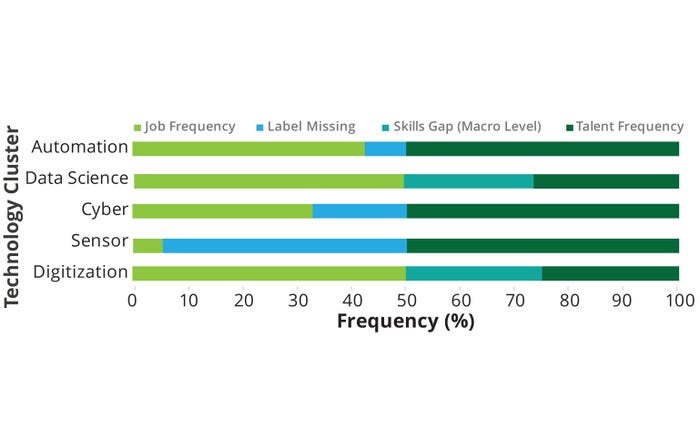
Figure 8: Skills gap — technology clusters.
The biomanufacturing field is evolving rapidly and generating vast amounts of data, creating a need for professionals with data-science skills (10). However, we found evidence of a skills gap in the industry when it comes to data science. The key attributes of that gap include data management/integration, data analysis/statistical modeling, ML/predictive analytics, data visualization/communication, and regulatory compliance/QC. Biomanufacturing generates data from a number of sources, including bioreactors, analytical instruments, and QC systems, with a requirement for professionals who can manage and integrate diverse data sets effectively to derive meaningful insights (52). Those include skills in data acquisition, data cleaning, data integration, and database management.
Data scientists with knowledge of statistical techniques, multivariate analysis (MVA), experimental design, and regression analysis can analyze and interpret complex data sets effectively to identify patterns and correlations for optimizing processes, improving product quality, and enhancing efficiency (46). ML techniques have significant potential in biomanufacturing for predicting process outcomes, optimizing parameters, and identifying anomalies. In addition, data scientists need to possess strong data-visualization and communication skills so that they can present complex data to nontechnical stakeholders. Proficiency in tools such as Tableau and Power BI software, with the ability to create intuitive visualizations, will be crucial to bridging the gap between data analysts and decision-makers (8). Finally, biomanufacturing operates within a highly regulated environment, and data scientists need to be familiar with regulatory requirements and QC practices. Such experts should understand validation procedures, compliance standards, and data-integrity principles to ensure that data-driven insights comply with regulatory guidelines and support QC processes.
Figure 9 illustrates a skills gap by job title. Titles were obtained for each job and profile post, and the most common titles were sorted based on their frequency in each category. In addition, we analyzed the total number of job titles and specific profiles. Our gap analysis compared the frequency of each skill in the supply and demand data (see above). That provided an indication of current biopharma 4.0 skills gaps at the job-title level, which translates to the fact that experts in the current talent pool are insufficient to satisfy bioindustry 4.0 demands. Figure 9 illustrates our assessment of the current biomanufacturing job titles, suggesting a skills gap in relation to validation, process development, and automation engineers. “Agile” is in high demand for numerous positions, with agile coaches and project managers evidently lacking in expertise.
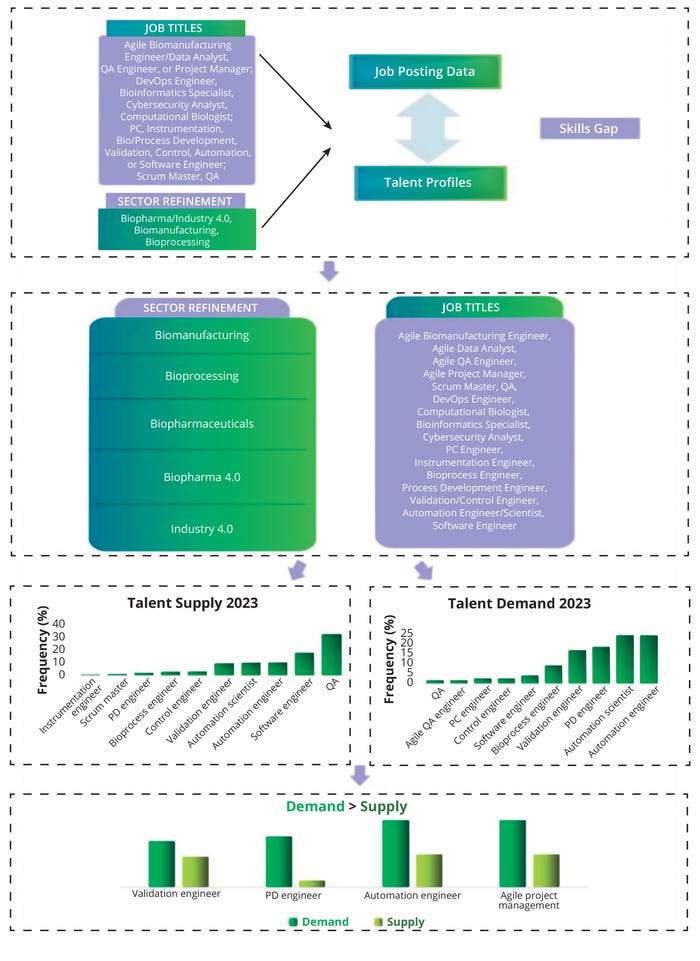
Figure 9: Skills gaps by biopharma 4.0 job title; QA = quality assurance, QC = quality control, DevOps = software development and information technology operations, PC = process control, PD = process development.
Discussion
The biopharmaceutical workforce market assessment presented herein provides a general analysis of emergent trends related to employee skills and expertise needed in the biomanufacturing sector, highlighting potential skills gaps associated with the automation, data-science, sensor, and cyber technology subdivisions. Industry and educational stakeholders can use our results to prepare the workforce for current and future talent demand through adapted recruitment and retention strategies as well as educational and training pipelines.
Current and Emerging Trends in Biomanufacturing Expertise: As predicted by unique job postings, the aggregate US demand for biopharma-4.0–correlated jobs has increased by 42% since 2018 (22). Rapid advancements in biomanufacturing technologies through biopharma 4.0 and an increasing focus on process optimization, data-driven decision-making, automation, and digitalization have created a growing need for individuals who can leverage those technologies effectively (8). Evidence suggests an increasing demand for data scientists, process engineers, and automation specialists as biomanufacturing companies strive to adopt the related biopharma 4.0 principles and technologies (3).
Specifically, our analysis of required expertise and specific skills revealed that Python, structured query language (SQL), and R are the principal programming skills in demand for the data science technology segment, with R accounting for the greatest talent deficit. Potential employees who are competent in Python, SQL, and R will be favored in current and future biomanufacturing industry hiring. In addition to those programming-language requirements, data analytics and processing tools play a key role in the data-science technology segment.
As job requirements evolve, biomanufacturing personnel need to acquire new expertise. Our future research will incorporate a more comprehensive analysis of the emerging data-processing skills requirements, including databases/platforms such as Kafka, Hadoop, Spark, and Cassandra software packages. That analysis will incorporate data management, real-time streaming, and scalable storage solutions. Additionally, cloud service platforms such as Microsoft Azure, Docker, and Amazon Web Services (AWS) could be the next set of tools to be included in future analysis.
Many of the skills and expertise recognized in our study have been present in other industry workforces, but only after the 2010s has data science gained popularity in the biomanufacturing industry (21). Upskilling of employees to provide robust data-science skills and attraction of proficiencies in programming, data science, and ML thus are critical to the biomanufacturing sector as it transitions to industry 4.0 (53). Our report on the skills deficit identified herein includes significant recommendations for company talent recruitment strategies, allowing organizations to upskill their employees and offer education and training opportunities. Such efforts would encourage the biomanufacturing industry to address its widening skills gap in the process of automation and digital transformation. Identifying critical gaps also would ease the transformation of traditional educational curricula in the biopharmaceutical industry toward a modern curriculum, with a focus on data and analytics.
Future Trends in Skills and Expertise: AI and ML have become increasingly valuable in the biomanufacturing field because of their potential to enhance process efficiency, QC, and decision-making (52). Proficiency in data analysis is a key skill for modern ML. It includes the ability to clean, preprocess, and analyze large data sets generated from different sources such as sensors, instruments, and production systems. Strong statistical knowledge is essential for identifying patterns or anomalies in data and then extracting meaningful insights (54).
Similarly, understanding and applying ML algorithms will be fundamental for biomanufacturing professionals. Professionals will need to possess knowledge of techniques involved in supervised learning (e.g., classification and regression), unsupervised learning (clustering and dimensionality reduction), and reinforcement learning. Familiarity with different algorithms such as decision trees, support vector machines, neural networks, and deep-learning architectures will be valuable for developing predictive models and optimizing manufacturing processes (46).
AI and ML skills are essential to optimizing biomanufacturing processes that use data-driven approaches to identify process bottlenecks, improve yields, reduce variability, and enhance efficiency. Employees need to be proficient in implementing optimization techniques such as genetic algorithms, particle-swarm optimization, and Bayesian optimization to fine-tune process parameters and achieve optimal production outcomes (26).
Finally, building accurate predictive models is a key skill within biomanufacturing that involves developing models to forecast product quality, predict process failures, estimate production yields, and optimize resource allocation. Professionals will need to be adept at selecting appropriate features, training models using historical data, and evaluating model performances to ensure reliability and generalizability (46). As the biomanufacturing field continues to embrace AI and ML technologies, professionals with such skills will be in high demand. Continuous learning and keeping updated with advancements in AI and ML techniques will be essential to leveraging these technologies effectively for biomanufacturing processes.
Insights for Planning Ahead
The emergence of industry 4.0 and the need for associated skills presents both opportunities and challenges for the biomanufacturing industry. Organizations must recognize the importance of nurturing these skills within their workforces to remain competitive and innovative in a rapidly changing landscape (32). Collaboration between industry and academia, robust training programs, and a culture of continuous learning are essential to addressing the current skills gap and ensuring that a skilled workforce will exist for the future of the biopharmaceutical industry (55). Biopharma 4.0 is reshaping the industry and driving the need for new specialized skill sets (8). Professionals with digital literacy, data-analytics expertise, automation/robotics knowledge, regulatory-compliance understanding, and adaptability will be well equipped to navigate the challenges and leverage the opportunities offered by biopharma 4.0. Embracing such skills will enable biopharmaceutical companies to thrive in an era of innovation and disruption (56).
We have investigated how automation and data-driven technologies have emerged to become established in the biomanufacturing industry. This study identified a skills and expertise gap in the current biopharmaceutical workforce. Data come from the JPA and PD modules in the Lightcast database, triangulated with LinkedIn and industry data. In addition, we created a unique analytical framework to compare the skill and knowledge requirements of the industry with what its available talent pool can offer. Analysis of the skill requirements confirmed that biopharma 4.0 roles require multidisciplinary expertise, and results indicated that many biomanufacturing employees are deficient in data-science and automation-related skills for biopharma 4.0. Furthermore, our analysis also highlights that the workforce needs enhanced programming skills to prevent expertise deficiencies between current job requirements and available workforce skills.
Considering the rise of industry 4.0 principles in biomanufacturing, companies might face several key obstacles to matching talent demand and supply. The imbalance stems predominantly from an absence of industry 4.0 skills in biology-related postgraduate education and available industry training. Although biopharma 4.0 training programs do offer exciting opportunities, the challenges described above need to be navigated by change management. Proactively addressing those challenges will help training programs effectively equip professionals with the skills and knowledge they need to succeed in the biopharma 4.0 era.
The future of training will be characterized by continuous learning, microlearning, blended approaches, virtual and augmented reality, data-driven analytics, personalized pathways, industry–academia collaboration, and lifelong-learning initiatives. By embracing those advancements, the biopharmaceutical industry can equip its workforce with the skills to thrive in a dynamic landscape and drive innovation in drug development. We have identified and illustrated gaps in skills and domain knowledge topics for major technology clusters specifically linked to the emergence of biopharma 4.0. The implications of this research could be useful to employees, with recommendations for skills upgrade in accordance with the emerging and future requirements. In addition, insights provided herein could be useful for designing data-science–related curricula for postgraduate biomanufacturing programs and training providers.
We anticipate a plethora of difficulties to arise within the biopharma talent supply chain as adoption of industry 4.0 increases. Growing technological complexity and broad adoption of digital innovations are prompting changes to requirements for new employees, with biomanufacturers requiring increasingly more diverse, technical, and scientific skills. Transitioning to an automation- and digitalization-driven operating model designed to increase connectivity and adaptivity in business operations will demand expertise not solely in the science of biopharmaceutical development, but also in its driving technologies.
Limitations of Research: Our investigation has some limitations. The data are a representative sample of the biopharmaceutical labor market and but are not necessarily comprehensive. Moreover, technical and operations roles have been emphasized in this study, whereas managerial level jobs are underrepresented. Future research could benefit from considering a broader range of job levels. Lightcast job analytics data can provide valuable insight into employment trends and skill demands; however, Table 3 lists some associated limitations to consider.
Table 3: Limitations of research.
Future Research: We believe that stakeholders will gain valuable insights into the biomanufacturing skills gap by conducting research in related areas, thus informing policy decisions and developing targeted interventions to address the highlighted challenges. Ultimately, the industry’s aim is to build a skilled and diverse workforce capable of driving innovation and growth in biomanufacturing. Our future research into skill gaps will focus on several key areas to help us further understand and address the challenges associated with expertise shortages in the sector (Table 4).
Table 4: Future research.
Acknowledgments
This report is based on work supported by Evolution Search Partners Ltd. and the University of Dundee’s doctorate in business administration (DBA) postgraduate program. All opinions, findings, conclusions, and recommendations expressed are those of the authors and not of the University of Dundee, University College London, or Evolution Search Partners Ltd.
References
3 Li G, et al. Data Science Skills and Domain Knowledge Requirements in the Manufacturing Industry: A Gap Analysis. J. Manufac. Sys. 60, 2021: 692–706; https://doi.org/10.1016/j.jmsy.2021.07.007.
8 Joshi H, Patel J. A Literature Review on Emerging Trends in Adopting Industry 4.0 Within Pharmaceutical Industry. Technology, Agility and Transformation: Emergent Business Practices. Shah T, Bhatia M, Shome S, Eds. Allied Publishers: Bengalore, India, 2023: 115–129.
10 Pokhriyal P, Chavda VP, Pathak M. Future Prospects and Challenges in the Implementation of AI and ML in Pharma Sector. Bioinformatics Tools for Pharmaceutical Drug Product Development. Wiley: Hoboken, NJ, 2023: 401–416.
21 Kumar V, Srinivasan V, Kumara S. Smart Vaccine Manufacturing Using Novel Biotechnology Platforms: A Study During COVID-19. J. Comput. Inform. Sci. Eng. (4) 2022: 040903; https://doi.org/10.1115/1.4053273.
22 Beckwith J, et al. The Talent Enigma in Digital Biomanufacturing. BioProcess Int. 21(1–2) 2023: 16–19; https://bioprocessintl.com/business/careers/the-talent-enigma-in-digital-biomanufacturing.
23 Yang C-T, et al. Big Data and Machine Learning Driven Bioprocessing: Recent Trends and Critical Analysis. Biores. Technol. 372, 2023: 128625; https://doi.org/10.1016/j.biortech.2023.128625.
25 Zhang G, Yang Y, Yang G. Smart Supply Chain Management in Industry 4.0: The Review, Research Agenda, and Strategies in North America. Ann. Oper. Res. 322, 2023: 1075–1117; https://doi.org/10.1007/s10479-022-04689-1.
26 Whysall Z, Owtram M, Brittain S. The New Talent Management Challenges of Industry 4.0. J. Management Develop. 38(2) 2019: 118–129; https://doi.org/10.1108/jmd- 06-2018-0181.
32 Bisschops M, Cameron L. Process Intensification and Industry 4.0: Mutually Enabling Trends. Process Control, Intensification, and Digitalisation in Continuous Biomanufacturing. Subramanian G, Ed. Wiley VCH: Weinheim, Germany, 2022: 209–229; http://dx.doi.org/10.1002/9783527827343.ch7.
33 Dieste M, Sauer PC, Orzes G. Organizational Tensions in Industry 4.0 Implementation: A Paradox Theory Approach. Int. J. Produc. Econ. 251, 2022: 108532; https://doi.org/10.1016/j.ijpe.2022.108532.
45 Pandey M, Singhal B. Blockchain Technology in Biomanufacturing: Current Perspective and Future Challenges. Blockchain Technology for Emerging Applications: A Comprehensive Approach. Islam SK, et al., Eds. Academic Press: Cambridge, MA, 2022: 207–237; https://doi.org/10.1016/C2020-0-03280-9.
46 Sarmadi A, Hassanzadeganroudsari M, Soltani M. Artificial Intelligence and Machine Learning Applications in Vaccine Development. Bioinformatics Tools for Pharmaceutical Drug Product Development. Chavda V, Anand K, Apostolopoulos V, Eds. Scrivener Publishing: Beverly, MA, 2023: 233–253; https://doi.org/10.1002/9781119865728.
47 Richter J, et al. Digital Twins in Bioprocess Engineering: Challenges and Possibilities. Chem. Ing. Technik. 95(4) 2023: 498–510; https://doi.org/10.1002/cite.202200166.
48 Rathore AS, Thakur G, Kateja N. Continuous Integrated Manufacturing for Biopharmaceuticals: A New Paradigm or an Empty Promise? Biotechnol. Bioeng. 120(2) 2023: 333–351; https://doi.org/10.1002/bit.28235.
49 Katina PF, et al. Advanced Manufacturing Management: A Systematic Literature Review. Sustainability 15(6) 2023: 4702; https://doi.org/10.3390/su15064702.
50 Gerzon G, Sheng Y, Kirkitadze M. Process Analytical Technologies: Advances in Bioprocess Integration and Future Perspectives. J. Pharmaceut. Biomed. Anal. 207, 2022: 114379; https://doi.org/10.1016/j.jpba.2021.114379.
51 Allenby MC, Woodruff MA. Image Analyses for Engineering Advanced Tissue Biomanufacturing Processes. Biomaterials 284, May 2022: 121514; https://doi.org/10.1016/j.biomaterials.2022.121514.
52 Rathore AS, et al. Artificial Intelligence and Machine Learning Applications in Biopharmaceutical Manufacturing. Trends Biotechnol. 41(4) 2022: 497–510; https://doi.org/10.1016/j.tibtech.2022.08.007.
53 Otokiti AU, et al. The Need to Prioritize Model-Updating Processes in Clinical Artificial Intelligence (AI) Models: Protocol for a Scoping Review. JMIR Res. Prot. 12, 2023: e37685; https://doi.org/10.2196/37685.
54 Pazhayattil AB, Konyu-Fogel G. An Empirical Study To Accelerate Machine Learning and Artificial Intelligence Adoption in Pharmaceutical Manufacturing Organizations. J. Gener. Med. 19(2) 2023: 81–91; http://dx.doi.org/10.1177/17411343221151109.
55 Cazeri GT, et al. Training for Industry 4.0: A Systematic Literature Review and Directions for Future Research. Brazilian J. Ops. Produc. Management 19(3) 2022: 1–19; https://doi.org/10.14488/BJOPM.2022.007.
56 Dutta P, et al. The Individual and Integrated Impact of Blockchain and IoT on Sustainable Supply Chains: A Systematic Review. Supply Chain Forum 24(4) 2023: 103–126; http://dx.doi.org/10.1080/16258312.2022.2082851.
Corresponding author Jason Beckwith, PhD, is managing director of Evolution Search Partners Ltd in Glasgow, UK, and a researcher in the department of business at the University of Dundee; 44-7899 950002; [email protected]. Paul Rooney, BSc, is a research consultant, and Gregor Adams is a research assistant with Evolution Search Partners Ltd. William Nixon, PhD, is an emeritus professor, and Stavros Kourtzidis, PhD, is deputy associate dean of research in the department of business at the University of Dundee. Stephen Goldrick, PhD, is an associate professor in the department of bioprocess engineering at University College London. The authors report no declarations of interest.
You May Also Like