Data Management in the Supply Chain
October 1, 2008
Adopting an effective strategy for data and knowledge management throughout the drug development and clinical manufacturing lifecycle is key to maintaining a competitive edge. Significant challenges face each organization seeking to improve efficiency in this area, and they can mostly be attributed to the complex nature of pharmaceutical drug development.
Managing both data and knowledge is complicated by the different groups, sites, and partner organizations involved with developing and manufacturing a new drug product. To further compound the problem, a significant proportion of data is most often stored in Microsoft Excel spreadsheets, paper-based systems, and disparate data silos, which makes location of information a particularly labor intensive chore. Under these conditions it becomes extremely difficult to extract any of the value locked within development data.
Novel IT solutions break down existing barriers and help significantly improve communication, data visibility, compliance, and operational efficiency across all development activities. An effective data management strategy can leverage the latest IT technology to seamlessly manage scientific innovation. Compliance and business performance can be handled in one integrated solution, helping organizations to adopt a quality by design approach that facilitates continuous improvement and ultimately reduces the cost and risk of delivering a new drug product to the market.
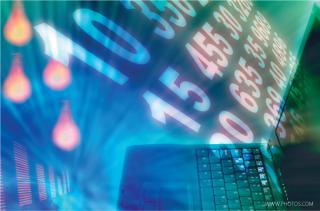
Figure 1: ()
WWW.PHOTOS.COM
From Development to Manufacturing
Moving a drug product through the development phases and into manufacturing generates vast amounts of data, which have to be married with all the regulatory documentation required in the preparation of a master drug file (MDF), new drug application (NDA), and standard operating procedures (SOPs). Data management during process design–optimization and the compilation of regulatory documentation is in most cases a manual process.
Pharmaceutical and biopharmaceutical organizations need to pull together all the scientific process data generated during process design with the validation and other regulatory documentation that are prerequisites for successful registration of new drug products.
Core Challenges
The following data management challenges are experienced by many pharmaceutical and biopharmaceutical organizations when generating data during the process design and optimization phases.
Different Data Formats: Because of the complex nature of process research and development, data are generated by several different groups such as analytical development, upstream development, and downstream development throughout the process development lifecycle. Typically, Microsoft Word and Excel documents hold scientific process data in folders on validated servers, and paper notebooks follow sign-off procedures and are manually archived.
Multiple Locations: Separate data sources typically contain information as varied as instrument output held on a file system on a network, analytical data captured and stored in silos, SCADA or Historian information, and LIMS-based databases. Because data sources are often spread across multiple locations, organizations cannot easily retrieve knowledge from their data and often fail to exploit the full value of this important asset.
That lack of cohesion also means that the rich context of development data is often lost, eliminating the chance to mine back into it at a later stage and retrieve meaningful associated information that could reveal the implications of potential process changes or answer critical business questions.
Report Generation: Generating reports is often frustrating and time-consuming because data are stored in multiple applications and in disparate systems. When reporting results internally — and in the case of contract manufacturing organizations (CMOs) — to client organizations, reports are manually compiled from each data silo and then stored in another on a validated server. Researchers are faced with copying and pasting from multiple paper sources, Word documents, or Excel files involving several error-prone transcription steps that introduce a large element of risk into the reporting process.
The results of a 2005 industry survey by PharmaManufacturing.com of development groups in 104 pharmaceutical organizations yielded the following conclusions:
Five hours a week is spent looking for data to prepare for reports.
Almost 20% of the time, needed data cannot be found.
More than 8% of studies have to be rerun because data are not accessible or cannot be retrieved.
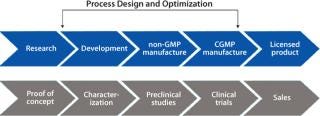
Figure 1: ()
Sharing and Communicating: Scientists often work on different parts of a process (fermentation, downstream processing, analytical development) and have to collaborate across different unit operations to pull the process together. Currently many organizations lack a reliable and accessible method of communicating and distributing collaborative information to departments involved in the process lifecycle.
Sharing information is also difficult between groups such as QA, QC, and manufacturing. Both biopharmaceutical and pharmaceutical organizations that partner with CMOs to outsource some or all development operations experience problems in sharing and transferring data, potentially across a number of global sites.
Compliance
Tracking changes made to data is almost impossible. For example, changes made to the value of a cell when transcribing data into a spreadsheet cannot be detected, presenting a big challenge when trying to prove who edited and authenticated such record changes. Regulatory compliance requires the identification of changes and the person responsible. This is an overarching consideration for organizations when trying to address data management issues.
Quality by Design
The FDA’s current good manufacturing practice (CGMP) for the 21st century and process analytic technologies (PAT) initiatives are currently significant considerations for most biopharmaceutical and pharmaceutical organizations. CGMP encourages the adoption of quality by design, a best-practices approach that integrates quality and risk management into development and clinical manufacturing.
When data are on paper and in scattered file sources, associated contextual information such as QC results and QA documentation may be lost or extremely time-consuming to compile. This makes it difficult to leverage internal data and knowledge to build quality into a process early in the development lifecycle. For example, if a validated process needs to be changed, researchers are faced with a potentially time-consuming search through paper records, databases, network files, and data silos to ascertain the implications of a change from both process science and quality standpoints. This means that right now, it is very difficult to adopt a true continuous-improvement–led approach to manufacturing and development that follows the philosophy of CGMP for the 21st century.
The PAT initiative, encouraging a risk-based and science-based approach for ensuring patient safety, emphasizes cost reduction and improved production efficiency through real-time process monitoring. PAT means that organizations must characterize their processes more than ever before by collecting development and contextual data and fully examining the implications of any deviations. Again, today’s lack of easily retrievable contextual process data from development through to manufacture hinders adherence to PAT and QbD guidelines, both of which aim to foster a culture of doing things right the first time.
All of these issues highlight the major data management challenges facing the pharmaceutical and biopharmaceutical industries today as they not only strive to successfully and efficiently move a new drug through the development lifecycle, but also to simultaneously drive the improvements in business performance, science value, and compliance required to maintain a competitive advantage. Organizations need to implement an effective data management strategy that allows information to be more efficiently compiled, searched, and made available to support better risk-based decisions.
The Data Management Solution
A compliant solution for integrating, capturing, analyzing, searching, and reporting process data can address common data management needs within the biopharmaceutical industry. The key points that a data management solution must provide to meet the challenges we have outlined so far are as follows.
Ability to Capture Any Data Type: A data management solution should offer the ability to capture the wide range of data formats that need to be captured throughout development including images, text, tables, forms, and content from Microsoft Office documents (spreadsheets and word processing documents). Instrument data should be easily captured directly from external systems, such as fermentor data, LIMS results, and HPLC and process chromatography controller data, along with content from cell line, equipment, SCADA or Historian, and protocol databases. The result of integrating these core process data is a fully searchable comprehensive electronic development history record supporting a process from early development to clinical production to technical transfer into commercial manufacturing. Once a commercial process is established, this development history record forms a support platform that enables continuous improvement.
Improved Communication Between Groups, Sites, and Sponsors: Task-flow tools allow a number of different types of communication, ranging from a simple sign-off request to a technology transfer of an electronic history record between development and clinical production. Vital components in a data management system, taskflow tools simplify and improve the efficiency of communication between users and departments helping in the transfer of information and processes between groups, sites, and sponsors.
Integration of Existing Data Capture Tools, Instruments, and Data Silos: Integration of tools and data repositories that organizations are already using within the development environment, both onsite and offsite is essential. This allows users to bring enterprise data together in one environment for analyzing, searching, and reporting so they can be effectively used to support decision making.
Standardized and Compliant Data Capture, Manipulation, and Analysis Tools: Standardized capture and analysis ensures consistent process development data and other contextual process data enabling accurate comparisons between all process development knowledge. Adherence to SOPs is essential in the biopharmaceutical and pharmaceutical industries. The ability to create robust and structured data capture templates that force a user to follow required workflows or SOPs when capturing and entering data can ensure data standardization. By enforcing standardization, a data management system improves data accuracy and ensures that an organization can be confident in its data quality.
Dynamic experiment templates that link to, for example, equipment or approved supplier databases, allow process engineers to more easily design with the end in mind. Organizations also are able to take more control over the development stages, helping to facilitate implementation of platform technologies in commercial and pilot manufacturing and ensuring a “right first time” approach.
Ease of Use with Simple Navigation and Organization of Data: To gain full adoption, a data management solution has to be easy to migrate into from existing systems. Simplicity of use is a must. Populating a record should be a straightforward drag-and-drop operation regardless of file type, with the ability to import and view file contents even if the application of origin is not on the user’s computer. This is important for ensuring that valuable record contents will remain viewable and unaltered irrespective of any changes to existing local or enterprise software such as a change in HPLC vendor.
Ability to Find Answers to Both Scientific and Business Questions: Full-text search capabilities and simplified reporting tools enable users to mine back through current and previous data to extract high-value knowledge. This information can be put directly into a report, saving time that can thus be spent developing new processes and adding value back into the organization.
Improved Knowledge Transfer and Knowledge Management and Reuse: Reporting templates that allow structured content to be added by drag and drop or automatic population simplify the process of creating weekly progress, comparative analysis, and technology transfer reports. Communication through task-flow tools also can be used to compile electronic history records into a package that can be transferred to another group, such as pilot manufacturing, for comparative analysis or technology transfer reports.
Improved Compliance: Built-in electronic signatures and witnessing must be integral to every data management system, with validation and auditing features that allow full compliance with 21 CFR Part 11. Process data linked to compliance data improves audit efficiency. However, more exploratory research requires a solution that has the ability to operate in a hybrid mode supporting both GxP and non-GxP business rules. Fully searchable electronic audit trails combined with full version control provide powerful assurance of the validity of all data and allows organizations to keep total control over all potential IP assets.
Ability to Find Answers to the “Who, What, When, Where, How, and Why” of the Development Lifecycle: When development data are linked to product and process data throughout its lifecycle, contextual information can be searched and mined as effectively as development data. A solution that retains every piece of data and knowledge generated around a process and follows that process all the way through development and into manufacturing represents a powerful unified hub of searchable information that facilitates process optimizations and other process investigations.
For example, if a given sterilizing filter is specified in development, and this filter has to be changed as the process transfers into manufacturing, then a user can mine back through supporting data to learn why that filter was selected in the first place and find the quality documentation that supported the initial selection. This helps reveal what the implications of changing the filter would be in terms of process, compliance, and validation burdens. And it enables the users to evaluate whether a filter change should be implemented, giving increased flexibility as a process moves into manufacturing.
A Continuous-Improvement–Led Approach and Enablement of Better Risk-Based Decisions Throughout Development and Manufacturing: The data management solution outlined so far fosters a more efficient continuous-improvement–led approach to drug development and manufacturing in line with the FDA’s CGMP guidelines, the benefits of which are reduced manufacturing costs, improved speed to market, and potentially less frequent regulatory inspections. Such a data management system would allow users to mine back into development data for comparative analysis and deviation tracking purposes to qualify and fully understand characterized processes. By enabling this characterization of processes, data management can guarantee the integrity of data from an early stage and help organizations to follow the FDA’s PAT initiative.
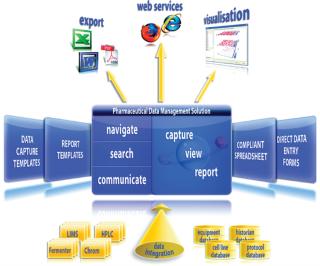
Figure 2: ()
Knowledge (Management) Is Power
There is a clearly defined need for a development lifecycle data management solution flexible enough to meet the requirements of all groups within drug and process development and clinical manufacturing. The key requirements are to improve efficiency, communication, compliance, and knowledge management. The ideal solution would provide data integration, capture, analysis, and reporting for process development and clinical manufacturing operations in one compliant portal.
The benefits of implementing such a solution would be
contracted development lifecycle — increased speed to market with higher project throughput
better risk based decisions
better process understanding and characterization (PAT and QbD)
improved development efficiency and project capacity
improved process optimization and responsiveness
more efficient workflows.
By improving data and knowledge capture and accessibility, this solution would dramatically improve productivity while enabling users to very simply answer who, what, when, where, how, and why questions throughout the development lifecycle. This would help reduce cycle times and improve the success rate for new drug molecules. Such a system allows both business performance and scientific innovation to be managed in harmony, providing a robust platform from which to realize the benefits of driving development operations into the 21st century.
You May Also Like